Today’s ecommerce ecosystem is fast integrating cutting-edge technologies and the power of artificial intelligence to redefine the way shoppers search for and discover products. In a world saturated with options, customers demand personalised experiences that captivate their attention and meet their unique needs to previously unforeseen levels. That’s why AI ecommerce product selection is now the most critical element there is; essential because it delivers the greatest returns. The revolution has begun.
That’s where AI steps in, revolutionising relevant product selection communications – emails, and discovery like never before. This revolution is significantly driven by user research, data capture, analysis and application of that data in one. It is understanding the nuanced needs and behaviours of each online shopper, and tailoring unique product selections to these insights.
Every individual on this planet is unique, and what might have been perceived a while ago as unfathomable impulse purchases, can now be revealed to be individual likes, dislikes, needs and aspirations. What’s more, AI now offers ecommerce retailers an immense opportunity, to new and staggering heights through the adoption of such facilities. Hyper-personalisation software is becoming not only essential but obligatory, as consumers cotton on to brands that understand and appreciate them.
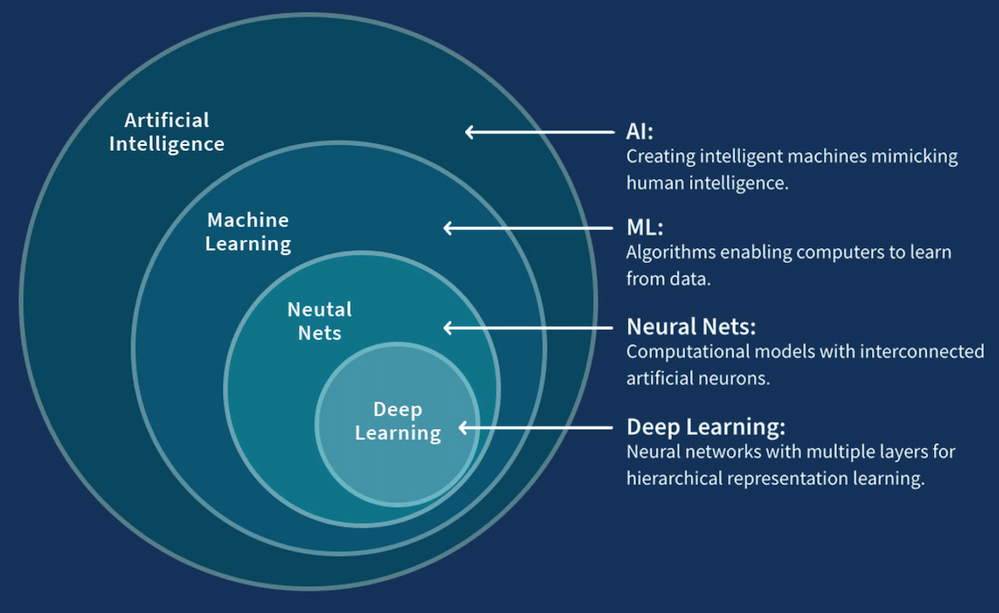
What is Product Selection and Discovery?
Product search, where customers actively look for specific items they intend to purchase. Is now almost irrelevant, when you can already identify what the consumer is most likely to buy both next and when, and then ensure it is presented to them at the relevancy of that item becomes paramount. Individualised product selection does not involve them entering specific keywords or phrases in the search bar anymore. If you’re still relying on that then be warned that the more work you make the customer do, the greater the demonstration that you have no idea who they are, let alone what they want. This is tantamount to capitulating your tenure as their preferred supplier.
Product discovery was once defined as stumbling upon products they might like, even if they weren’t actively searching for them. Again in the last couple of years, this has been flushed down the pan into oblivion by a solution devised to present the exact product that individual wants as they visit your site. The distinction apparent to successful CMOs universally, is that if the consumer only visits your site to see what you want to offer them, then your peers will beat you to it.
Hence hyper-personalisation of email marketing is becoming the critical mainstay of the next generation of market-stimulating solutions. It offers both low-hanging fruit in the form of what every dictates each consumer has revealed in their shopping, but also previously unforeseen selections are now captured too. Patterns not inhibited by anything anyone else bought – herd culture, “people who bought that also bought this alternative”.
The journey to effective hyper-personalisation is a continuous process. It requires ongoing data collection, analysis, and optimisation to ensure that each person’s personalised experience remains relevant and valuable to that customer. The key is to keep the customer at the centre of all efforts, using insights derived from data to drive decision-making and strategy.
Hyper-personalisation in the context of a customer-centric view requires businesses to respond in real time and also be proactive. This dual strategy enhances customer loyalty and engagement.
The first mechanism, “Real-time interactions and responsive actions,” (Forbes) responds to live data, for instance, notifying a customer about a price drop for a product they’re interested in. The key to this strategy is timing, relevance, and avoiding overly intrusive interactions.
The second mechanism, “Action-initiated communication,” triggers communication based on specific customer behaviour, such as sending a personalised email for an abandoned shopping cart or re-engaging an inactive customer with a special deal. the distinction is in the ROI. But triggered solutions fall short of the power of AI individualisation by 20:1, as it never gets ahead of the curve, presenting products before your peers know they’re interested.
Finally, the third mechanism, “Predictive and generative engagements,” leverages generative AI to anticipate future customer behaviour and create content accordingly. It can generate highly personalised content that caters to a customer’s future needs, such as a personalised shopping guide or a promotion highlighting a brand’s sustainability efforts, for a customer who frequently shops for sustainable products. These mechanisms, together, provide a highly personalised customer experience, augmenting customer engagement and boosting conversion rates.
In the dynamic landscape of ecommerce, AI technology is reshaping product search to meet the evolving needs of customers. Let’s explore how AI-driven technologies and strategies are optimising search and driving customer satisfaction in the context of the ecommerce industry.
Natural Language Processing (NLP)
NLP can help gain a deeper understanding of your customers. This can reveal valuable insights about their needs, preferences, and behaviours, which can be used to create more targeted and personalised marketing messages. For instance, a business can use NLP to analyse the process to arrive at a purchase decision on your website. This can reveal common themes and trends, such as what customers like or dislike about the business’s products or services, which can be used to inform future marketing messages.
NLP can also be used to personalise customer interactions. For example, businesses can use NLP to generate personalised responses to customer queries or comments. This can make customers feel valued and appreciated, which can increase their loyalty and likelihood of making a purchase. NLP to send personalised marketing messages to each customer. For instance, a business could use NLP is now often used to analyse a customer’s purchase history but also their browsing history, and navigation, determining the greatest likelihood of purchase when a specific route is taken, which then enables sophisticated solutions, hyper-personalisation solutions, to send them an ever increasingly accurate personalised product recommendation based on this information.
Natural Language Processing (NLP) enables ecommerce platforms to understand and interpret the nuances of behaviour. With NLP, AI algorithms can grasp the context, intent, and sentiment behind the entire customer journey and lifetime, allowing for more accurate selection and naturally therefore results. This technology is particularly valuable in ecommerce, where customers often make their purchase decisions in unique and personal ways defining their unique take on what life (and you) have to offer. By employing NLP for semantic application, you can provide customers with highly targeted results, improving their overall shopping experience.
Differences between Statistical Modelling and Machine Learning
The biggest difference between statistics and ML is their purposes. While statistical models are used for finding and explaining the relationships between variables, ML models are built to provide accurate predictions without explicit programming. Although some statistical models can make predictions, the accuracy of these models is usually not the best as they cannot capture complex relationships between data. On the other hand, ML models can provide better predictions, but it is more difficult to understand and explain them. Admittedly where the data is vast, and perpetually updating, especially in frequent site visits or purchases, deep learning, a discipline within machine learning becomes the greater focus.
Statistical models explicitly specify a probabilistic model for the data and identify variables that are usually interpretable and of special interest, such as the effects of predictor variables. In addition to identifying relationships between variables, statistical models establish both the scale and significance of the relationship.
By contrast, ML models are more empirical. ML usually does not impose relationships between predictors and outcomes, nor isolate the effect of any single variable. Let’s go back to the consumer example. If we get the data of 20 million people with 100 clicks each, per site visit, and we mainly want to predict AOV and relevancy of individual SKUs, we may use a machine learning model as there are so many variables. We may not understand the relationships between variables and how the model makes sense, but what we are after is accurate predictions.
Essential components for achieving hyper-personalisation
- Data collection: This is the first step, and perhaps the most crucial. You need to collect detailed data about your customers. This is no longer demographic data but is transaction history, browsing behaviour, length of time viewing a product, repeat product viewing data, social media activity, purchase history, browsing history, search history, social media activity, cognitive dissonance analysis, and other online interactions. This data is then analysed using machine learning algorithms to create personalised experiences for each consumer.
- Data analysis: Once you’ve collected the data, it needs to be analysed to extract meaningful insights. This could involve identifying trends, preferences, and behaviour that can help predict future actions.
- Artificial intelligence, machine learning, and generative AI: AI, and ML are the engines that drive hyper-personalisation. These technologies can analyse large amounts of data, learn from it, and make predictions or decisions without being explicitly programmed to perform the task. Generative AI takes personalisation beyond reactive adjustments and actions, enabling businesses to predict and generate content tailored to anticipate future customer behaviour and preferences. This includes creating custom promotional offers, hyper-personalised product selection emails, or unique user experiences. By doing so, generative AI adds another layer of proactiveness to personalisation, significantly enhancing customer engagement and taking the personalisation aspect to new heights by adding a Generative Experience.
- Real-time decision making: Hyper-personalisation requires making real-time decisions based on the collected data and insights. This could be as simple as serving up a personalised product recommendation or as complex as dynamically tailoring the entire user experience.
- Customer journey mapping: Understanding and monetising the customer journey is essential to provide individualised personalised experiences at every touchpoint. This involves identifying the different stages customers go through when interacting with your brand, from the awareness stage to the purchase stage, and beyond.
- Security and privacy: As you’ll be dealing with large amounts of personal data, it’s crucial to ensure that you’re handling this data responsibly and complying with all relevant privacy laws and regulations.
- Testing and optimisation: Finally, continuous testing and optimisation are important. This involves regularly testing your personalisation efforts to see what works and what doesn’t, and making necessary adjustments to improve the customer experience. Adoption of already advanced technology is readily available through adoption via plugins from companies that do nothing else than enhance and deliver the greatest possible returns for you already.