The modern consumer has ever-changing preferences and to satisfy them brands are turning to AI hyper-personalisation strategies that help to increase customer engagement, boost loyalty, and ultimately drive sales. In this article, we will discuss the topic of AI-powered hyper-personalisation, highlight some remarkable ways businesses use it across different industries, and explore some of the tools and tips businesses can use to level up their individualisation game.
The success of modern businesses depends on their ability to keep customers engaged with hyper-personalised experiences that speak directly to their individual needs and interests. Despite noise to the contrary, compared to what they like themselves, consumers don’t care a damn about what anyone else likes. The exception is fashion, where wearing what’s popular, be it subconsciously wearing it, still haunts the market. Then again they need to be reassured about their purchase a human trait called cognitive dissonance.
The modern customer has ever-changing preferences. To satisfy these customers, brands are turning to AI personalisation, a technology powered by artificial intelligence, machine learning, and natural language processing. With the new AI-powered personalisation tools, brands are actively exploring how to increase customer satisfaction and engagement, boost loyalty, and ultimately drive sales.
What is AI hyper-personalisation?
AI hyper-personalisation refers to using AI technologies such as machine learning and deep learning to create curated and unique individual experiences for users of a given product or service. Using hyper-personalisation, brands aim for a better customer experience and satisfaction, which in return increases engagement, customer satisfaction, and conversion rates.
AI hyper-personalisation leverages the ability of machine learning to ingest large amounts of data, analyse it and produce insights. Using this data, algorithms can establish patterns and behaviours and make predictions about customer preferences and interests. Some of the data you can use include browsing data, interactions, reviews, and feedback. With this data, machine learning tools can build different profiles that correspond to different user preferences.
Based on the insights, businesses can then customise their marketing messages, content, product offerings, and recommendations to appeal to the unique needs of each of their customers. Avoid any assumption this has anything to do with segmentation, the bane of past marketing failures. AI hyper-personalisation is used across different industries, including ecommerce, social media, entertainment, social media, and technology.
7 impressive applications and examples of AI personalisation
AI-powered personalisation has countless applications across different industries. In this section, we explore how brands use hyper-personalisation AI and data from browsing, purchase histories, geographic location, online behaviour, demographic data, etc. to create experiences unique to individuals.
1. Product recommendation
The ecommerce market is highly competitive, with estimates stating that there are currently over 26 million ecommerce sites across the entire globe. For any business to survive in such a saturated market, it must tailor unique product offerings that attract customers, which is where recommendation systems come in.
AI hyper-personalisation in ecommerce is a perfect example of how AI has revolutionised an entire industry. With the advent of AI-powered hyper-personalisation, brands are moving away from the traditional rule-based recommendation systems that have been successful in the past. Traditional recommender systems are inflexible and assume customer preferences remain unchanged over time. They also have limited ability to handle data and complex relationships.
Using modern machine-learning capabilities, brands can now analyze various data points and recommend products a particular user will likely buy. For instance, brands can recommend products based on ratings and reviews, engagement, purchase history, similar customers, demographic data, or based on seasonal trends.
Many notable brands have already adopted AI personalisation in ecommerce. For instance, Amazon, an American multinational ecommerce platform, leverages Amazon Personalise, an ML-based recommendation system, to reduce abandoned carts by recommending products to users based on their preferences.
2. AI hyper-personalisation in ad-targeting
Customers are often bombarded with thousands of ads on web browsers and across social media platforms like Facebook. Sometimes these ads become annoying and creepy, pushing users to look for ways to avoid seeing them on their platforms. However, studies suggest that showing users ads that align with their interests is more likely to get them to take action.
Using AI, brands can analyse customer data, such as purchasing history, social media activity, location, demographic data, and other details to create personalised ads. A personalised advertising campaign is more likely to resonate with your audience, boosting engagement and conversion rates. Other metrics that brands can use to create more targeted ads include social posts, comments, likes, and shares.
Facebook is one of the companies that have an AI-powered ad targeting system that allows companies to target different audiences based on their traits. Some of the possible forms of targeting with Facebook ads include:
- location-based targeting,
- interest-based,
- behaviour-based,
- job title,
- education level,
- political views,
- connections,
- targeting based on demographics.
For instance, a user who constantly shares information about clothing is more likely to be interested in ads showing clothes.
Facebook is the leading ad-targeting platform, with over 2 billion active users and thousands of data points. With ad targeting, brands can narrow down their ad audience and increase response rates.
3. AI content personalisation
Most brands spend a big budget on SEO and content marketing to build a loyal audience. However, all these efforts and resources can be in vain if you keep showing your users generic content they don’t want to see. Using AI content personalisation, brands can now improve reach by showing users the content they want to see when they want to see it. AI content personalisation involves using machine learning to analyse data and determine the type of content that resonates best with a given audience. It is now all about relevancy.
Many brands have found AI content personalisation a great tool to engage customers and keep them returning to their sites. Warner Bros. Discovery, a premier global media and entertainment company, has succeeded with AI content personalisation.
Using Amazon AI Personalise Warner Bros. Discovery has customised movie and show recommendations for unauthenticated users. According to their VP of Architecture, Don Browning, the company saw a 14% increase in user engagement and a 12% increase in cross-brand engagement since implementing content personalisation.
4. Dynamic pricing
Dynamic pricing is a pricing strategy that involves adjusting the price of a service or product based on certain factors. AI personalisation can play a crucial role in making pricing decisions. Using machine learning, brands can analyse huge amounts of data about various aspects and make informed price changes in real-time.
Some of the data leveraged by dynamic pricing algorithms includes market trends, production costs, competitor prices, location, demographic data, and customer purchase behaviour.
There are many industries where dynamic pricing is typical, including the airline, eCommerce, hospitality, utility, and retail industries.
One familiar brand that uses dynamic pricing is Uber, a tech company offering mobility services. Using the Uber app, passengers can hail a ride and pay a fee to the drivers to get to where they want. When you request an Uber ride on a Friday evening, you’ll realize that the prices aren’t the same as on an ordinary Tuesday.
Uber uses a machine learning-powered surge pricing algorithm to determine pricing during peak hours. The algorithm recommends reasonable prices based on geolocation data, and demand forecasting to position drivers more appropriately, increasing customer satisfaction and profits.
Another example of dynamic pricing is in the airline industry, where the price of tickets changes depending on the time of the year, the popularity of the route, the number of seats left, and competition. For instance, on the flights below at Delta Airlines from JFK in New York to London Heathrow, the prices are slightly higher for flights leaving JFK at 9:30 pm compared to those leaving at 8:30 pm on a certain Friday.
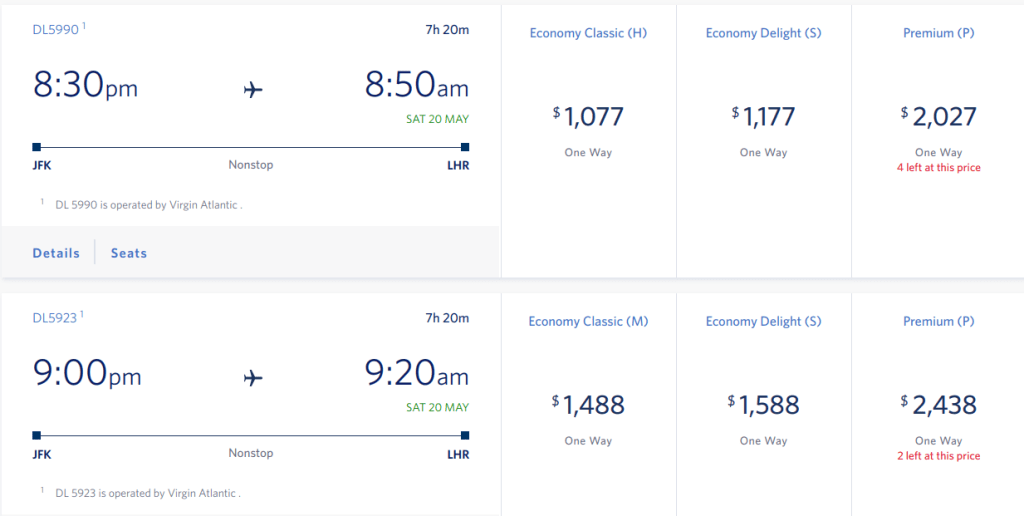
Through AI-powered personalisation in dynamic pricing, airlines can maximize profit, reduce churn rate, improve customer experience, and remain competitive.
5. Hyper-personalised messaging and emails
Modern customers are smarter and savvier and can easily know when you’re bombarding them with irrelevant messages that add no value. Brands must hyper-personalise their messaging or risk being labelled as spam and an audience and potential leads as a result.
To personalise communication, businesses need to understand their customers and audiences by evaluating different data points.
Through hyper-personalisation AI, brands can meaningfully engage with their customer through highly curated and contextual communication sent to the right audience at the right time. Personalised communication establishes trust, deepens relationships, and increases the willingness of customers to spend on your products.
Hyper-personalisation of AI and profiling customers into different personas annihilates the returns of old methods specifically segmentation when communicating via email. Instead, the focus is on delivering individual experiences businesses can apply throughout their customer communication relationship.
SwiftERM offers an AI hyper-personalisation solution installed as a SaaS. Downloaded from respective available platforms, it is believed to be currently the only wholly autonomous solution, thereby delivering the highest ROI in ecommerce marketing according to international research firms including Forrester, McKinsey, Statista, Bain and many more. The autonomy raises the game as cost-free from human involvement. More importantly, it ensures that each consumer receives details of the products pertinent to them, and not what a segment wants, or worse merely what a retailer wants to sell.
By ensuring the products are on the money and selection perfect, machine learning avoids errors and omissions that humans can’t help but do, and therefore the loyalty to the retailers grows exponentially.
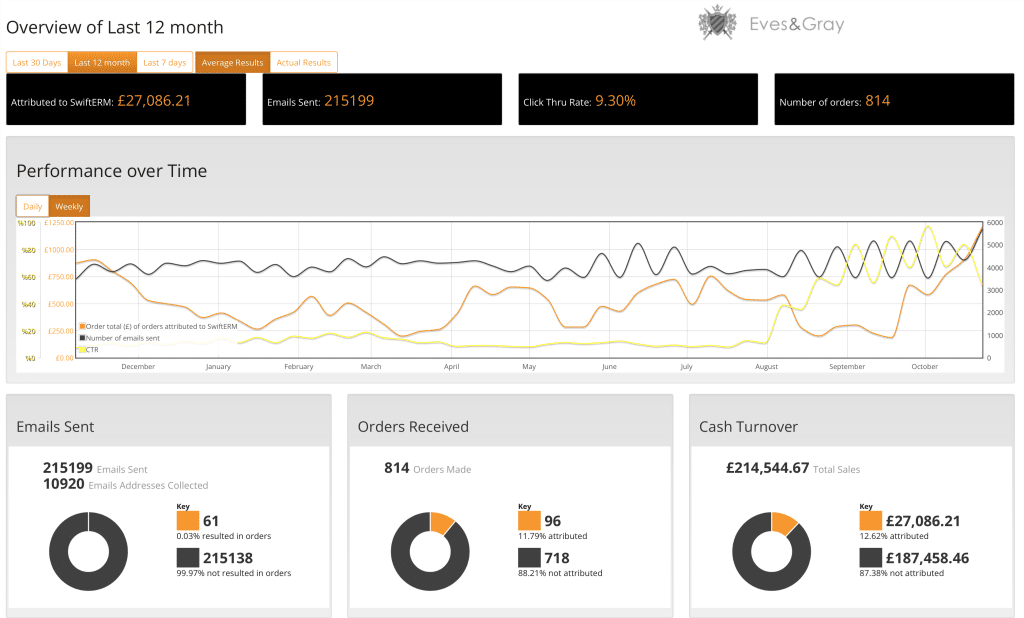
6. AI hyper-personalisation in dynamic websites
With most customers now relying on online information to make purchase decisions, businesses need to have a credible website to create brand awareness and establish credibility.
To provide a better and more personalised user experience, websites also need to be dynamic. Unlike static sites, dynamic sites change content after every user interaction or input. Through dynamic websites, users can also perform functions such as shopping, real-time chatting, and content sharing, functionalities not achievable through static sites.
Using AI personalisation, businesses such as ecommerce platforms can show users more relevant content based on data. This data can include a user’s past browsing, activity, location, and age, among other factors.
Nike is among the most popular brands in the world that leverage AI-powered personalisation to show users of their platforms products they’re more likely to be interested in. For example, if you have previously shopped for running shoes, Nike is more likely to recommend those to you.
Through the dynamic nature of its website, Nike is more likely to improve customer experience and satisfaction.
7. Hyper-personalised AI-powered chatbots
Chatbots have evolved exponentially in the past, from simple rule-based applications to efficient support systems that offer excellent customer support. The new chatbots are not only AI-powered but also can understand customer queries and create personalised responses. Here is how personalisation works in chatbots.
- Chatbots use Natural Language Processing to understand and interpret queries and respond appropriately.
- Chatbots now have contextual understanding and can recall past conversions providing a more personal experience.
- Using user data such as past purchase history, preferences, past interactions, and other relevant data chatbots create an individual user profile that matches individual unique characteristics.
- Continuous learning and adaptation: Chatbots learn in real-time as they interact with users and adjust their preferences accordingly, providing a more personalised experience.
- Using machine learning algorithms, chatbots can analyse huge amounts of data and identify trends and patterns in user behaviour.
Through personalised chatbots, brands can enhance customer experience, increase engagement, provide proactive support, collect valuable data, and provide 24/7 support.
Conclusion
Hyper-personalisation is by far the most powerful marketing element that businesses can leverage for growth. However, AI has revolutionised individualisation and how businesses engage with their customers. Using the power of AI hyper-personalisation, businesses can now deliver hyper-personalised experiences, increase customer satisfaction, and drive revenue growth. From hyper-personalised messaging, content, ads, recommendations, and AI-powered chatbots, AI applications keep growing by the day. However, before implementing AI hyper-personalisation, businesses need to have a clear goal and be conscious of any ethical implications.