This article focuses on the conception and use of machine-learning predictive personalisation algorithms reshaping consumer knowledge in ecommerce marketing. In the last years, specialised services have been increasingly using machine learning to predict consumer behaviour now being adopted by any size of company.
Predictive marketing revives the old dream of one-to-one, perfectly adjusted selling techniques, but at an unprecedented scale. So how do predictive marketing solutions change the way corporations understand their customers?
There is an ambivalence that characterises the promise of personalisation, i.e. algorithmic processes in which the precise adjustment of prediction to unique individuals involves the computation of massive datasets.
But looking at many algorithms in practice, there is commonly an actively embedding local preexisting assumption of consumer knowledge and de-personalisation mechanism, key to the epistemic and organisational success of predictive marketing. We believe that the algorithm, and their contexts, suggests new perspectives on algorithmic objectivity.
We will focus here on predictive algorithms, whose current momentum, 25 years after the first email marketing campaigns, perpetuates the digitalisation of marketing methods. Indeed, during the last few years, a new kind of algorithms, often labeled as “artificial intelligence” but more precisely belonging to the field of machine learning, has spread at an unprecedented scale. Their main feature is the ability to make statistical predictions from very large sets of heterogeneous, possibly unstructured data.
A customer’s interest in a given product, their risk of churn, or fraud, is predicted through algorithmic models that assign probabilistic scores to individual customers. These scores rely on data describing their past behaviours, whether collected from the web, or already held and stored in the companies’ internal databases, with the obvious intent to then apply them going forward.
Predictive personalisation as a disputed moral ground
Personalisation is the cornerstone of contemporary algorithmic solutions. What we buy, what we look at, how long we look at it, how many times we come back to it, both pre and post purchase. There are many more components of our everyday interaction with an ecommerce website and it’s communications, each increasingly dependent on algorithmic suggestions, supposedly tailored to fit our personal interests.
Personalisation for commercial uses has mostly been criticised by Big Data scholarship. Many authors see it as a mere cynical communication artifice, a fairytale vision used by marketers to dissimulate the algorithmic manipulation of consumers. In this perspective, personalisation would essentially mean a strengthened grip on individual behaviour and the optimisation of conversion rates.
This new marketing paradigm would thus now allows unprecedented control by companies over their consumers potential purchasing behaviour, through sophisticated algorithms and ever-increasing data volumes. Predictive marketing technologies therefore produce “self-grounded” representations of consumers, out of clustered data points of disembodied interests, behaviours, opinions and demographics.
Personalisation has become an essential moral underpinning of Big Data quantification devices, justifying practices, on the users’ side, but also on the part of developers and practitioners.
The perfect client: When the algorithm predicts too well
The post-campaign evaluation generated another inconsistency, when Predicto realised that the customers who actually purchased a policy were not the top-ranked ones: they did have a good ranking, but the top-scored customers proved to be surprisingly unresponsive to the company’s solicitations. This observation called for another bit of interpretative work.
Learning algorithms displace uncertainty in customer knowledge. In accordance with the historical ambition of market research, they quite considerably reduce the uncertainty surrounding the customer’s tastes and behaviour. They do, however, induce a new type of uncertainty, linked to the explanation of scores (black box effect), but above all to their interpretation. Contrary to symmetric critics and praises regarding machine learning algorithms, they do not produce a cold, seamless, and automated process of knowing and governing data points. Until the last moment, sadly humans often adjust the parameters and nature of algorithmic action on people.
What happens to the consumer in this process? Far from being dissolved within algorithmic computation, their taking into account constitutes both the horizon and the material support of a set of varied practices.
Organisational settings actively aim to embed the usual categories and perceptions of the consumer in the choice of analysed data, and in the types of questions for the algorithm to solve. The algorithmic predictions demands a specific interpretation work, or “casual theorising”, that reattaches them to “palatable” figures of the consumer and plausible courses of action. Predictive personalisation software (PPS) is just one example, in which highly likely imminent purchases are identified and emailed to the consumer before competitors know about it, or the moment is lost.
These moments, before and after the calculation, are key conditions of its possibility, success and performativity upon the social world. In other words, it is because data and algorithms are continuously readjusted to exogenous preexisting forms of knowledge that personalisation of entire databases can happen. Classical categorisations of the consumer are essential to the organisational as well as to the epistemic success (i.e. producing “useful” predictions) of predictive marketing.
Algorithmic modelling seems to be a mechanistic knowledge instrument, in which the automated abstraction of calculation should guarantee the elimination of human preconceptions and biases, and would thus produce more “objective” results than those generated by traditional market research.
Nevertheless, predictive personalisation in ecommerce marketing now delivers enormous return on marketing investment, through application of precise and enormous volumes of personal data on each consumer.
Further practical and academic articles on ecommerce marketing are available here.
SwiftERM is a Microsoft Partner company.
Article Classification
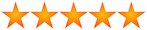