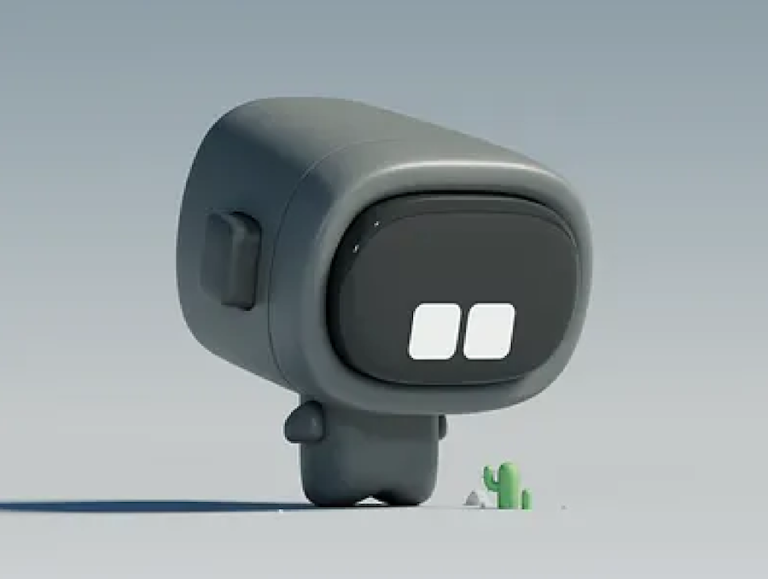
Harnessing AI-Driven Machine Learning to Revolutionise Ecommerce: Strategies for Success
Ecommerce is changing fast. More businesses now use AI to stay ahead. AI-Machine learning, a key part of AI, makes online shopping smarter. But with
Ecommerce is changing fast. More businesses now use AI to stay ahead. AI-Machine learning, a key part of AI, makes online shopping smarter. But with
While both hyper-personalisation and segmentation are critical strategies in email marketing, they represent different approaches to delivering tailored content to consumers. Understanding the distinction between
The transition from monolithic architectures to microservices has revolutionised deployments and team productivity, yet it has also brought about the tedium of daily repetitive tasks
AI has been around a while now and is a central topic in discussions around business and consumer technology, as well as in popular media.
Deep learning (DL) is rapidly becoming one of the most prominent topics in the realm of materials data science, with a quickly expanding array of
This week, DeepSeek created waves throughout the global technology sector as it surpassed ChatGPT in Apple’s application marketplace. This remarkable ascent has altered the dynamics
The Human Element in AI-Driven Ecommerce: Courtesy of The Harvard Business Review Reflecting on the wealth of data and trends presented in this report, one
You frequently visit your favourite ecommerce store, a significant part of this is how their emails resonate perfectly with your likes, wants, and needs. If
The intersection of vast data and artificial intelligence (AI) is paving the way for transformative shifts in how brands connect with their audiences. Conventional marketing
Hyper-personalisation is a marketing approach driven by data that leverages artificial intelligence (AI), machine learning, and extensive data analysis to provide bespoke content, products, and
Artificial Intelligence has evolved to the point where it can create both programs and written content. Currently, it serves as a generative AI development assistant
Photo by Thirdman from Pexels As new technological tools and platforms emerge, the healthcare sector must be the one most affected by such disruptions. New
What exactly is individualisation? How does it differ from personalisation? And why should it matter to your organisation? Advertising frequently focuses on specific target audiences –
People’s buying decisions might appear complex, but everyone follows certain steps to determine which products and services suit their requirements. Reflect on how you think
Your company’s main website is strong and efficient, catering to customers within your home country. Now, you aim to recreate these functionalities for a customer
Artificial Intelligence (AI) has transformed the landscape of predictive analytics, enabling companies to quickly process and examine enormous datasets. Through AI technologies like machine learning,
AI models hold significant benefits, especially when GPT collaborates with a search engine on an online shopping platform, it is likely to improve the shopping
In the fast-changing world of digital technology, hyper-personalisation has become a key tactic for crafting highly individualised customer experiences. Using sophisticated data analysis, artificial intelligence,