According to research, 4 out of 5 marketers believe that personalisation is essential to successfully compete today. Therefore, companies are gradually abandoning mass-marketing strategies—i.e. one message for everyone—in favour of the far more profitable – hyper-personalisation variety.
This allows them to be able to personalise customer experiences, web content, email product selection, offers and messages. Sadly if you are only just up to this stage, then you are already well behind and need to play catch-up significantly. We shall explain:
When it comes to personalising on-site marketing actions there are two methods of achieving this:
- Manual personalisation (governed by fixed, pre-set rules),
- Hyper-personalisation (based on flexible, machine learning algorithms)
What are the differences between the two and which is best suited to your business challenges? Are they exclusive or complementary? This article aims to provide the answers.
What is personalisation
Personalisation involves adapting an offer, message or content to an individual target. So before ecommerce sites can personalise, they need to know who they are addressing. Whether it’s manual or hyper-personalisation relies on the collection and processing of each visitor’s data to identify the needs of a target before offering them a relevant browsing experience or specific marketing action.
One example is Send Time Optimisation, in which the AI algorithm has identified when the specific individual is going to be abe most receptive the receiving their product selection, something impossible manually.
Manual personalisation
With manual personalisation, the targeting and triggering of your contextualised actions depend on rules (or criteria) that have been decided and set in advance.
Therefore personalisation is somewhat misinterpreted as up to only a few years ago what was believed to be personalisation, was merely segmenting, which is not personalisation, it still lumps people together, nevertheless is performed based on the real-time analysis of so-called “hot” data on the one hand—i.e. the data linked directly to the context of the visit in progress on the website (behavioural, contextual and technical)—and of “cold” data on the other (i.e. historic visitor data from your CRM, DMP, CDP, etc.).
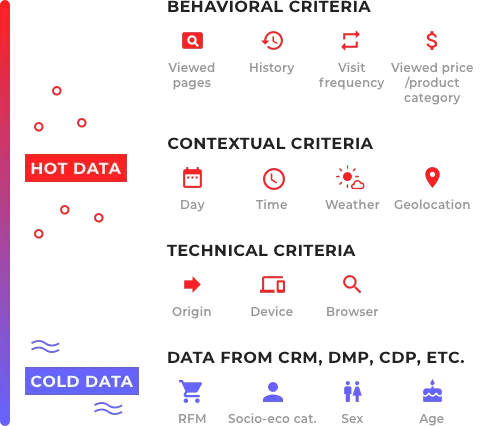
Once segmentation is complete brands can push on-site marketing actions to the different visitor segments of a website (for example, new visitors, customers for more than two years, customers using mobiles, cart abandoners, etc.).
Like yourself, would you enjoy being lumped in together with a bunch of other people if the attempt at personalising content to you made a distinction no more than all those who bought jeans in the last month? On the one hand fine, but no mention of brand, price point or colour. If you’re discerning enough to have bought Zegna 12MILMIL12 Wool joggers and your ecommerce retailers send you men’s loose fit Jacamo, would you feel the love?
Hyper-personalisation
As for hyper-personalisation, it doesn’t depend on rules that are static but is based on real-time analysis of all visitor data by artificial intelligence. This type of personalisation relies on an AI machine learning algorithm.
Machine learning is a branch of artificial intelligence which consists of feeding data to an algorithm, which then learns from it to make predictions. There are many distinctions between hyper-personalisation vendors to be aware of.
There are three key stages in its use:
1. Assign the algorithm a goal
The role of the algorithm is to measure the conversion probability of each visitor to your website in real time. The first step is therefore to establish which “conversion” is required. The conversion goal given to the algorithm can, for example, be the purchasing probability in a given price category, or even the probability that message A will convert a visitor more effectively than message B. But then go a step further as the algorithm also sees buying patterns, how long a purchase decision took, the criteria selection as illustrated by every impression, previous buying history, and what the purchase was complimenting. All elements that a site without hyper-personalisation intrinsic to its core don’t appreciate what its competitor is capable of taking away from them
2. The algorithm feeds on all visitor data within the data ecosystem
The algorithm begins its learning process using the hot and/or cold data that it is fed. It learns from the behaviour of the visitors to the website, searches for correlations between visitors and improves its capacity to determine each visitor’s conversion probability over time.
3. The algorithm identifies in real-time each visitor’s interest in specific marketing actions
The algorithm determines each visitor’s conversion probability and adjusts its actions accordingly (triggering contextual actions only when relevant). Such a solution could include the delivery of hyper-personalised product selection for each consumer on a perpetual basis from SaaS solutions such as SwiftERM. this Microsoft Partner solution, can either run as a stand-alone email solution or run alongside the likes of Klaviyo or Emarsys etc and deliver (according to McKinsey and Statista) as much as 20x the orders otherwise achieved. Effectively it is knowing what the consumer wants and when, then offering it to them.
How data science evolved from a manual to machine-learning model
In the field of data analysis and processing, rule-based manual personalisation systems work flawlessly, provided that all eventualities are known in advance by the person who sets the rules. In the past, smaller traffic volumes, less complex data and more standard customer journeys made the setting of these rules relatively simple. Today, with high volumes of complex and at times unstructured data, it is increasingly difficult to stick to a rule-based system. This is where machine learning comes in.
If we classify any kind of data by categories (A, B, C, D, E, etc.), a machine learning algorithm can learn, by itself, what defines the elements of each category, or indeed regardless of category, and process this data in real time. This evolving model is taking the lead in data science, but also in marketing and personalisation.
Manual personalisation vs. hyper-personalisation: should you favour one over the other?
Given that the algorithm improves and adapts its prediction capability in real-time and 24/7, its performance is always better than a human’s performance. This is particularly true if the hot data (behavioural or contextual visit data) plays a decisive role in identifying the optimal target or offering for your actions.
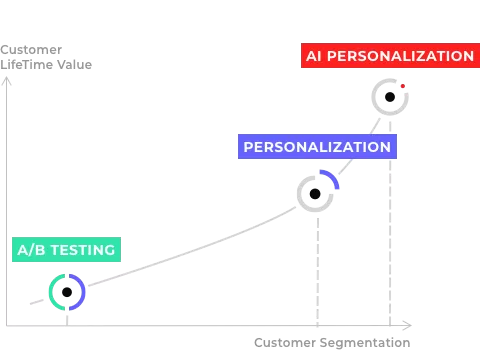
However, this doesn’t rule out the manual approach for actions where the target has been identified and has easily configurable characteristics (new vs old visitors, desktop vs mobile, etc.). However, bear in mind that with the growing number of personalisation actions being undertaken on a website, the manual approach can turn out to be painstaking and hard to orchestrate. The financial savvy will appreciate the cost implication in terms of staff can add a huge burden in addition to the required break-even as well.
One of the clear advantages of the hyper-personalisation approach is also that it enables you to automate actions and it can better manage data volume, complexity and variety compared to the human brain.