In the world of marketing, the hype around generative AI is at an all-time high. According to a recent BCG Group survey, global Chief Marketing Officers (CMOs) are bullish on AI’s potential to boost productivity and deliver a competitive edge.
Seventy per cent are already implementing AI-powered marketing, 19 per cent are testing it, and the top areas they’re looking at are hyper-personalisation (67 per cent), content (49 per cent) and sadly still -as they haven’t moved with the times market segmentation (41 per cent). But for most consumer brands, there’s a huge disconnect between what they’re hoping for and what’s happening. For marketers who want to deliver a seamless, magic customer experience, they need to understand that AI’s success depends on the quality of the underlying data it’s based on. If that’s not there, then the AI won’t work.
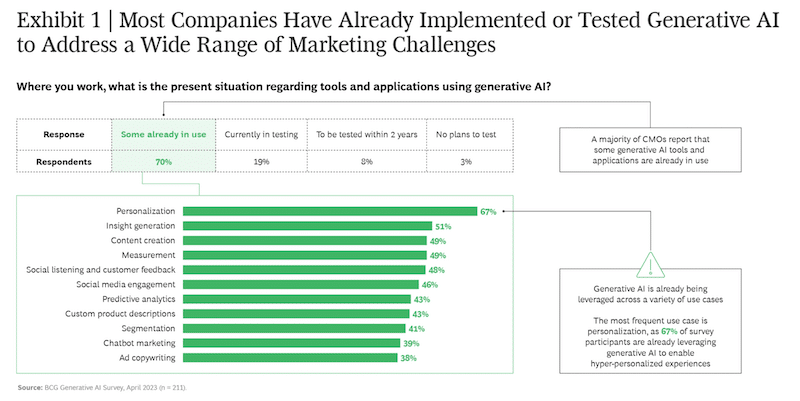
What happens if the quality of data is poor?
Let’s look a bit more closely at what an AI-powered marketing experience with poor data quality might look like: Let’s say I’m a customer of a generic sportswear and outdoor store and I’m about to embark on my annual winter skiing trip. I’m looking forward to enjoying the brand’s personal product selection AI, which I’m confident will provide me with a straightforward experience that’s simple and brings relevant AI-powered marketing to serve my needs.
I have a style, and strong brand loyalty, and appreciate a technical standard of sophistication reflecting my abilities, so I’m expecting the AI to suggest the most pertinent products for me. The problem is that the AI is building its responses based on information about me that’s been spread across the company’s various systems. Without a clear idea of who I am, it’s asking for some basic information it should already know, which I find aggravating, to say the least. I’d go so far as to consider paying a little more from another retailer I usually only reserve for specialist items as they charge most for their branded gear.
Because my information is so disparate, the concierge AI only has my name linked to an order from 2 years ago. That order was a gift, not a purchase. Without a complete picture of who I am, this AI hyper-personalised product selection software isn’t able to provide real-time insights and ends up sending recommendations that aren’t relevant. lt should already know about me. Other retailers use a choice of hyper-personalisation solutions that don’t just rely on purchase history, but the nuances and subtleties of my navigation, and the length of time I spend looking at a product, and then relate that to my previous purchases, to see where my thinking is leading.
In the end, this poor experience made me less interested in buying from this retailer, so I decided to go somewhere else. The reason for disconnected and impersonal generic AI is data quality. Poor data quality = bad customer experience.
Autonomous AI-powered marketing with clean data
Using the same analogy, but but this time where the AI hyper-personalisation uses, real-time data that has a full history of my experience with the brand from my first purchase, through reactions to emails they’ve sent me, to my last return. This time instead of waiting to go to the site to have what I most desire put in front of me, the communication from my retailer is perfect.
It knows what I’m after, I believe even what my proficiency level is, as it offers the most relevant products to me in an email of exactly what I need now. This hyper-personalised revolution, between the retailer and I, is respected as it’s a friendly professional relationship. It begins to build loyalty through this one-to-one experience with a helpful sales interaction. It autonomously links my shopping history and matches my past purchases with my current shopping requirements.
The concierge responds to my product views and purchases and provides a hyper-personalised set of solutions to complement my existing gear along with direct purchase links. The AI then learns again from the selections offered that I don’t look at or buy. They could be still of interest but may have already been purchased elsewhere or put off because of cost. Either way, I appreciate it notes that lack of interest and complements my further service with an infinitely finer selection next time.
Solving the data quality problem
Unified customer data is first and foremost of the utmost importance for building an effective AI strategy. The challenge is that customer data is large and complex, with most consumers having at least 2 email addresses, moving 11 times in their lifetime and using an average of 5 channels (or 12 channels if they’re millennials or Gen Z).
There are several tried and true methods for unifying customer data, such as rules-based and data-fuzzy matching. However, these methods are inflexible and fall apart when data doesn’t match perfectly. This can lead to an inaccurate customer profile, missing a large portion of a customer’s lifetime history with a brand and not taking into account recent purchases or changes in contact information.
The best way to create a unified data foundation is to use AI models (a variation of AI for marketing rather than generic AI) to identify the relationships between data points to see if they belong to the same person, with the same level of nuance and flexibility as a human but at a much larger scale. When your CRM tools can leverage AI to connect every touchpoint of the customer journey (from first interaction to final purchase and beyond) including loyalty, email, site data, etc, the result is a complete customer profile that shows you who your customers are and how they engage with your brand.
How data quality in generative AI drives growth
For the vast majority of marketers, the same set of AI tools are available, so the data you input will be your competitive advantage. Data quality powering AI offers advantages in three key areas:
- Customer experiences that stand out — more personalised, creative offers, better customer service interactions, a smoother end-to-end experience, etc.
- Operational efficiency gains for your teams — faster time to market, less manual intervention, better ROI on campaigns, etc.
- Reduced compute costs — better-informed AI doesn’t need to go back and forth with the user, which saves on racking up API calls that quickly get expensive
As AI tools for marketing evolve, they bring with them the promise of maximising the level of ROI for marketing through hyper-personalisation, a level that customers now expect in their favourite shops, but on a much larger scale. But that won’t happen by itself — brands need to give AI tools and accurate customer data to make the AI magic happen. Some solutions are already available to help you, that do it all for you.
Optimism and Confidence Far Outweigh Worry
The survey revealed that optimism and confidence in AI’s future are at an all-time high. When asked to name the areas of greatest opportunity, optimism in AI hyper-personalisation AI, (70%) is listed by the CMOs as their top solution requirement. What’s more, this optimism isn’t just spreading in ecommerce. It’s spreading across every country and every industry.