Deep learning is a method in artificial intelligence which teaches computers to process data in ways and patterns inspired by the function of the human brain. It is already a reality proving extremely beneficial to ecommerce and digital retail.
From autonomous product recommendations to enhanced hyper-personalisation, it is now changing the face of digital retail so you know exactly how it could give your business the most competitive edge it has ever known.
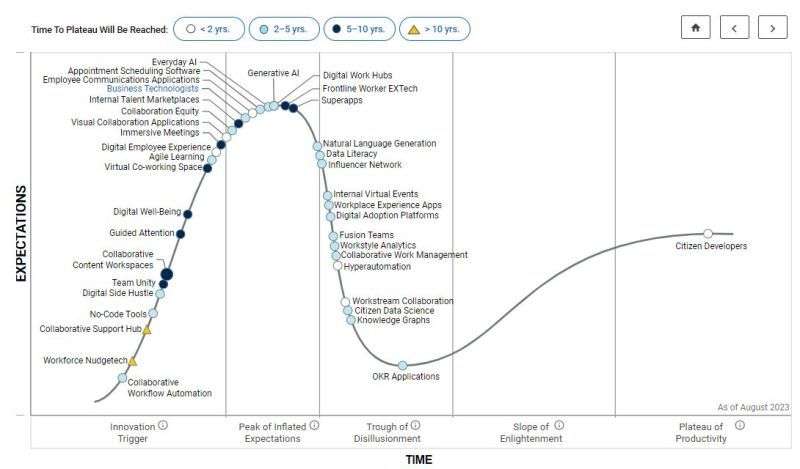
When new technologies make bold promises, how do you discern the hype from what is commercially viable? And when will such claims pay off, if at all? Hype Cycles provide a graphic representation of the maturity and adoption of technologies and applications, and how they are potentially relevant to solving real business problems and exploiting new opportunities. Hype Cycle methodology gives you a view of how a technology or application will evolve, providing a sound source of insight to manage its deployment within the context of your specific business goals.
How do you use Hype Cycles?
Clients use Hype Cycles to get educated about the promise of an emerging technology within the context of their industry and individual appetite for risk. Should you make an early move? If you are willing to combine risk-taking with an understanding that risky investments do not always pay off, you could reap the rewards of early adoption.
Is a moderate approach appropriate? CMOs who are more moderate understand the argument for an early investment but will also insist on a sound cost/benefit analysis when new ways of doing things are not yet fully proven. Should you wait for further maturation? If there are too many unanswered questions around the commercial viability of an emerging technology, it may be better to wait until others have been able to deliver tangible value.
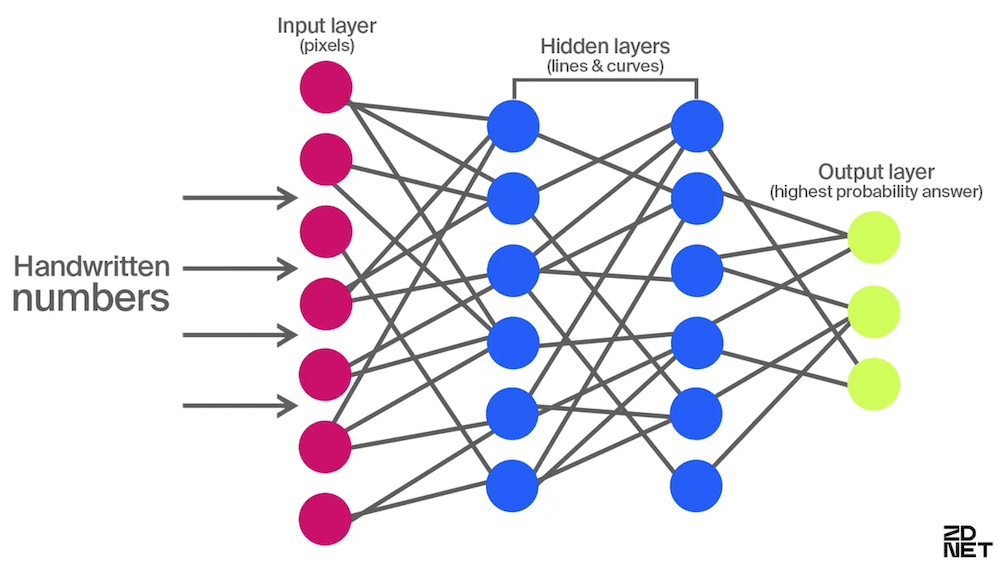
Deep learning is a class of machine learning algorithms that uses multiple layers to progressively extract higher-level features from the raw input. For example, in image processing, lower layers may identify edges, while higher layers may identify the concepts relevant to a human such as digits or letters or faces.
Improved Product Recommendations.
Deep learning does not make it possible to read minds, obviously, but it comes close enough. That’s thanks to the accuracy with which it can predict which products customers are likely to buy. It does this by not only watching the patterns within both product purchase selections but also views, clicks, abandons et al. In addition, it also looks for navigation patterns, as, these can differ as much as taste and opinion, culminating in an understanding of a consumer at different times.
Improving product selection recommendations by analysing customer data – things like the customer’s browsing history, purchase history, and even social media activity – is one of the most significant ways deep learning algorithms have revolutionised ecommerce. But AI is part of artificial intelligence, not something that the megalomaniac proprietor then leaps in to change, just because it suits their whim. leave your personality at the door when entering, this solution works autonomously in return it delivers a 20-fold increase in ROI, according to Forester and McKinsey.
Crunching this data means those algorithms have a keen understanding of what a customer is looking for. That makes it easier for websites and mobile applications to suggest suitable products tailored to their needs. The autonomy delivers those products selected to the consumer in the form of emails, rather than waiting for them to choose to visit your site. the personal product selections make the option to purchase from you many-fold more likely than spending your hard-earning marketing budget merely on CRO.
A smarter recommendation engine for ecommerce doesn’t just make your life easier. It makes the whole shopping experience more relevant for your visitors. Stop thinking about loyalty and start thinking about relevance. They take some of the research out of product research and put the shopper on the fast track to making a purchase. It’s a win-win.
Dynamic Pricing
Your prices aren’t chiselled in stone. Demand, competition, and customer behaviour all play a part in adjusting what you charge and whether that price is higher or lower for any product on any given day. To stay a step ahead of the competition, dynamic pricing adjustments are essential. If your site or app offers a product at a noticeably higher price point than a competitor, it’s easy to see how that’s going to hurt your bottom line.
Deep learning is already being put to work across the retail landscape to implement dynamic pricing strategies. Amazon for example uses dynamic pricing to optimise product prices as frequently as every 10 minutes. The addition of this tactic led to a 143% increase in profits between 2016 and 2019. Deep learning analyses relevant factors in nanoseconds and adjusts pricing in real-time to maximise sales opportunities across the web and mobile shopping apps. Handling this task manually would be a herculean effort and leave you wide open to over or under-charging.
Offer Optimisation
We just saw that thanks to sophisticated algorithms and neural networks, deep learning can predict customer behaviour and preferences with incredible accuracy. This insight means you can effortlessly tailor your offers to each customer, increasing the chances of recording a sale and making it much less challenging to build customer loyalty. That last point is especially important right now because brand loyalty recently hit an eight-year low.
Let’s put this into context. Growing sales from an existing customer is cheaper than winning a new customer. But it’s like the Wild West out there. Your customers are being bombarded with tempting deals from rivals desperate to make their sales.
Deep learning analysis of a customer’s past purchases, browsing history, and social media activity can determine what products they are likely to buy in the future, so you don’t have to navigate that battleground alone. Your audience engine* means you’ll know what your customer wants even before they do. No matter who they are and what they are shopping for, you can serve tailored, hard-to-pass-up suggestions and offers, at any time.
Starbucks is one example of a brand doing offers optimisation well. Users of its rewards app are often sent offers, tailored to each person’s buying habits, to encourage them to return to the store. Offers could be anything from buy one get one free, free in-store refills and extra reward points on orders placed before a certain period.
Driving Deep Insights About Customers and Products
You’ve got this far so it should be obvious that deep learning is changing the game for ecommerce and digital retailers. It provides businesses of all sizes with useful tools to stay ahead of the competition and deliver an exceptional customer experience.
Deep learning can also help retailers extract valuable insights from customer data collected from websites and mobile applications to continue that cycle. This data is invaluable to understanding the behaviour, preferences, and needs of customers. It also makes it much easier to identify significant patterns and trends in customer data which can be fed into product design and pricing strategies.
It is no use to you however unless you have a cost-effective means to integrate it simply into your tech stack, and more importantly strategy. technologies such as hyper-personalisation solutions, among which the distinctions between the top 30 providers are considerable., make this simple, as this 100% autonomous solution gathers data from day 1, and returns product selections immediately for each consumer, thereby allowing even the humble start-up to make profits from AI deep learning from the second it goes live.
SwiftERM is a Microsoft Partner Company.