CLV is the value a customer contributes to your business over “their” entire lifetime buying from your company, rather than anything to do with actual their lifetime, as they may change loyalty many times.
The main methods of calculating CLV are split between historic and predictive CLV:
- Historic CLV (Good indication of CLV) Simply the sum of the gross profit from all historic purchases for an individual customer.
- Predictive CLV (Great indication of CLV) A predictive analysis of previous transaction history and various behavioural indicators which forecast the lifetime value of an individual. As long as the equation is accurate, this value will become more accurate with every purchase and interaction.
Why is Customer Lifetime Value (CLV) important?
Customer lifetime value is one of the most important metrics in your tool belt if you’re an ecommerce retailer.
We all know that it’s more costly to acquire new prospects than to retain existing ones – thus extending your CLV is central to a healthy business model and customer retention strategy.
Don’t believe us? Here are five reasons why employing CLV as a central metric is vital if you want to increase profitability, retention and overall ecommerce success.
1. Generate real ROI on customer acquisition
CLV helps you focus on the channels that give you the best, most profitable customers. You should be optimising your marketing channels in terms of the lifetime value a customer contributes to your brand, rather than the gross profit on the initial purchase.
You are therefore trying to maximise your customer lifetime value about your cost of customer acquisition (CLV: CAC).
Focusing on CLV will change the economics of your customer acquisition strategy. Suddenly you can pay a lot more to acquire a customer because you are not held back by the profit generated from a single purchase but from the purchases made over a lifetime with your brand.
Information about your customers with the highest CLV (known as your VIP customers) will also give you insight into exactly who you should be targeting in terms of demographic.
Factoring CLV into your strategy is a recipe for success, and will leave all your less data-driven competitors in the dust while you’re busy ruling and dancing in your ecommerce disco (which you threw because you rule).
2. Enhance your retention marketing strategy
The value of a marketing campaign (for example, one aimed at turning your one-time purchasers into repeat customers) should not just be valued on the instant revenue it drives. It should be valued in terms of what impact it had on the average CLV of the segment of customers you are targeting.
How did it alter the trajectory of CLV over time for an average customer? To calculate this, you’ll need accurate predictive analytics so that you can see how predicted CLV is influenced by different marketing actions.
3. Create more effective messaging, targeting & nurturing
You can segment your customer base by CLV so that you can improve the relevance of your marketing with more personalised messaging. *Although this has now been overtaken by AI hyper-personalisation.
A useful variable to use here would be the types of products you market to your customers from different segments.
4. Improve your behavioural triggers
By organising data into natural groupings (or clusters) you can discover the behavioural triggers that incentivised your best customers to make their first purchase.
Once you’ve taken a look at your beautiful results, you should be trying to replicate this behaviour with your prospective customers to turn them into first-time purchasers.
5. Improve output from customer support
Focus your time on giving special attention to your most valuable customers. Never forget Pareto’s handy little principle: 20% of your customers generate 80% of your revenue.
Using CLV to identify your most valuable customers will help you decide where to direct your customer service resources. Paying attention to your most valuable (and profitable) customers will help you push up margins, at the same time as fostering strong relationships through better service with your most important segment.
Customer lifetime value calculation
Historic CLV
This is simply the sum of the gross profit from all historic purchases for an individual customer. Sum all gross profit values up to transaction N where transaction N is the last transaction a customer made with your store. If you have access to all your customer transactional data you can calculate this in Excel.
CLV (Historic) = (Transaction1+Transaction2+Transaction3…+TransactionN) X AGM
(AGM = Average Gross Margin)
Calculating CLV based on net profit ultimately gives you the actual profit a customer is contributing to your store. This takes into account customer service costs, cost of returns, acquisition costs, cost of marketing tools etc. The issue with this is that it can be highly complex to calculate this on an individual basis, especially if you want the figures to constantly be up to date. Gross margin CLV will still give you great insight into the true profitability of your customers to date.
Predictive CLV
Predictive CLV algorithms try to obtain a more accurate value of CLV by predicting the total value a customer will eventually give to your store over their entire lifetime.
CLV is always the NPV (net present value) of the sum of all future revenues from a customer, minus all costs associated with that customer.
In practice, this can be hard to achieve due to the requirement for up-to-date discount rates. There are numerous ways to calculate a predictive CLV that vary wildly in complexity and accuracy however we will focus on a couple of examples here. Simple and Detailed.
Simple
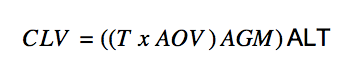
Where:
T = Average monthly transactions
AOV = Average order value
ALT = Average Customer Lifespan (in months)
AGM = Average gross margin
Let’s call the above equation gross margin contribution per customer lifespan (GML).
Detailed
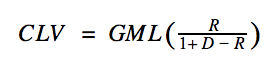
Where:
R = monthly retention rate
D = monthly discount rate
Be aware that these models will never be exactly right, they are just forecasts.
Predictive techniques…are always limited to the horizon of our models’ predictive accuracy and confidence.
Having said this the more tailored your CLV equation is to your specific industry the more accurate it will likely be. The best models are highly accurate. It is essential to do these calculations to get a real understanding of how valuable your customers are, the more accurate you become the more powerful your marketing can be.
One important factor the previous equation misses out on is the ongoing costs to your business for retaining a particular customer. You would need to calculate this to get a net value for CLV.
In addition, the most complex predictive models make increasingly more accurate CLV figures based on how a specific individual continues to interact with your store. Taking into account both interaction and transactional information.
As we know, not every individual is the same—some are a lot more valuable than others; as you gather more data about an individual, you can determine with increasing accuracy what sort of individual they are likely to be: High, Medium, Low value etc.
Highest returning CLV in marketing
If you’re just venturing into the possibilities using personalisation, there’s no better time to start. Recent studies show that hyper-personalisation has gone mainstream.
Subscribers want it and expect it. It helps boost email engagement. And, it delivers such a significant increase in sales, its omission is now the most heinous crime against marketing. The greatest impact is in email marketing.
Marketers using hyper-personalisation track both website and email response activity perpetually. It is an autonomous solution, that requires zero human input whatsoever, and it makes returns 20x greater (according to McKinsey, Forbes etc) in comparison to any of the the examples above and omnichannel marketing combined. It removes not only the cost of human involvement but their errors and omissions too.
Dynamic content becomes an integral element in all predictive personalised emails, which smashes ROI. Using dynamic content with personalised emails packs a powerful punch. For those researching hyper-personalisation, it is essential you choose autonomous software with zero human interaction involvement to achieve the greatest returns or you could spend time and money, wasted on an inferior solution.