Top trends in data and analytics technology and practices can help anticipate change and transform uncertainty into opportunity. Data and Analytics (D&A) leaders must factor these trends into key investments that drive new growth, efficiency, resilience and innovation.
Overview
Opportunities
- “Thinking Like a Business” allows the D&A function to focus on adopting a proactive approach to delivering value while also accepting the responsibility of “owning” the implications of its activity.
- Moving “From Platforms to Ecosystems” thinking allows the entire organisation to be both more agile in delivering immediate capabilities and to ensure a consistent and holistic view of both the technical architecture and the organisational context of its activity.
- “Don’t Forget the Humans” is a necessary rallying cry for organisations that may have become too “data-centric or technology enamoured” and lost touch with the engagement of their employees and their broader responsibilities to the organisations and society.
- AI has seen a move to wholly autonomous solutions, delivering a significantly higher ROI as a consequence. Early adoption offers opportunities to steal a march on competitors and win significant trances of market share, especially in the distinction of ecommerce personalisation.
Recommendations
Data and analytics leaders must:
- Define their role as leader of a business centre delivering key insights to decision-makers across the organisation. Act more like an internal consulting company with its P&L than a business support function that represents a cost centre to the rest of the business.
- Plan their D&A strategy in the context of ecosystem thinking where each element impacts and is impacted by the whole. This means investments in D&A teams must work with their Enterprise Architect colleagues to ensure D&A capabilities are factored into a consistent broad technical architecture as well the balance of risk and reward D&A investments can bring.
- Plan on engaging with stakeholders across the organisation to understand the best approach to drive D&A adoption (which will not always just mean more and better analysis and insights but also align with human psychology and values).
What You Need to Know
The mission of data and analytics is clarifying from one of managing data resources and creating analytic insight to delivering value. This need to deliver provable value to the organisation, and to do so at scale, is driving a variety of trends across the data and analytic function.
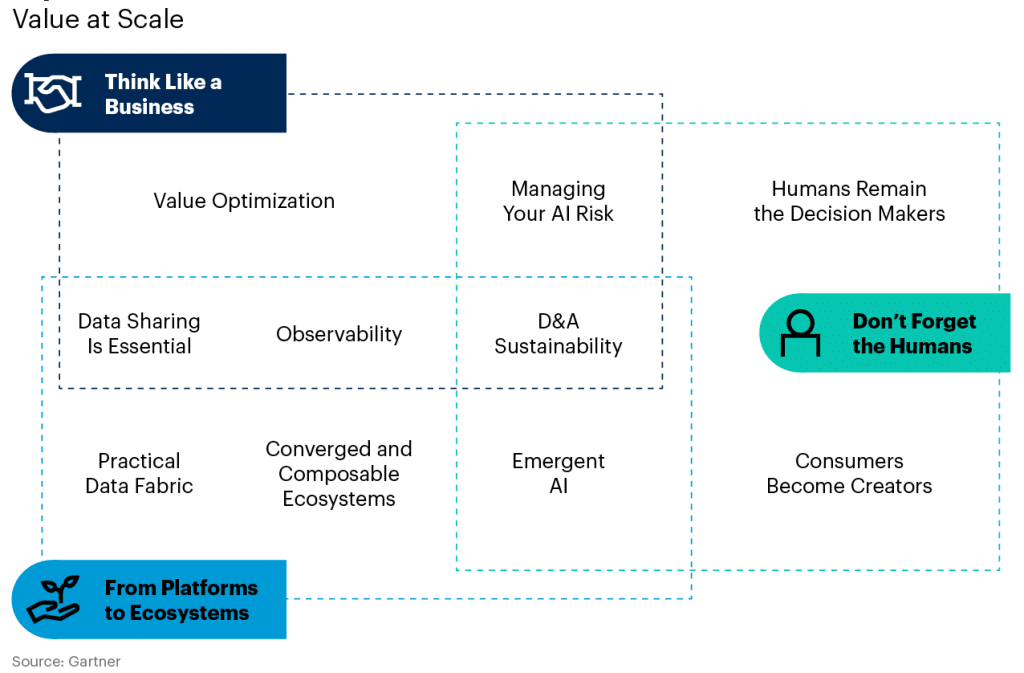
These trends can be broadly grouped into three themes:
- “Think like a business” is a requirement for focusing on the value delivered to the organisation rather than the production of insight.
- “From platforms to ecosystems” is the adoption of a more agile and all-encompassing approach to thinking about how data and analytics integrate into the rest of the organisation.
- “Don’t forget the humans” is the recognition that, although organisations are increasingly automated, data and analytics (especially AI) are better aligned with people rather than used as a substitute.
Theme 1: “Think Like a Business”
The data and analytics strategy of a think-like-a-business leader identifies how the data and analytics team will deliver maximum value to the organisation in a “competitive landscape” against disconnected D&A initiatives across the organisation, external substitutes or a “no data” mindset.
This shift in mindset has four key implications:
- Be proactive, not reactive: Leading CDAOs are proactive about driving awareness, understanding and usage of data and analytics across the organisation. They are not “order takers” waiting for requests for a new dashboard or predictive model.
- Devise an approach for selling data and analytics to the rest of the organisation: Framing the value of data and analytics in the language of the “customer” as a “product” that will drive growth and innovation, manage risk and reduce costs to the rest of the organisation (their mission-critical priorities) is essential.
- Balancing the cost of delivery with the value delivered: D&A leaders have a variety of mechanisms to deliver value (for example, developing skills in-house versus working through consulting partners), and part of thinking like a business is to understand the trade-offs implied by these different approaches. D&A leaders must understand the cost implications of different technological approaches, not just to minimise costs, but to enable a discussion about which approach delivers maximum ROI.
- Own responsibility for data and analytics assets and outcomes: Thinking like a business means acting as part of a value chain or ecosystem and also accepting responsibility for the consequences of your actions. Not only do D&A leaders need to ensure the adoption and usage of their insights, but they also need to act to avoid improper use of the assets they are creating.
Trends reflecting this theme:
- Value optimisation drives a focus on business impact.
- Managing your AI risk drives thinking about the risks and rewards of adopting new technologies.
- Data sharing is essential and reflects the need for a full understanding of the environment.
- D&A sustainability acknowledges the double-edged sword that analytics represents.
- Observability drives the focus on understanding the cost drivers of analytic activity.
Theme 2: “From Platforms to Ecosystems”
Increasingly strategic use of data and analytics and business need for agility is driving a shift from siloed and disconnected components in data and analytics platforms to integrated D&A ecosystems. This is enabled by market convergence across data and analytics capabilities and improved service composability. But a D&A ecosystem is not just a network of technical platforms. As a socio-technical system, it should also encompass considerations such as:
- When technology is a substitute for human workers and when it is a complementary enhancement
- The role that humans and AI play in the broader social and environmental ecosystem.
This shift in focus has three key implications:
- Increasingly comprehensive and integrated end-to-end architectures make adding new capabilities more seamless and less costly: D&A capabilities that are less costly and that have a less complex architecture require little or no integration of products and cloud services. This lowers the barriers to adoption and increases the viability of marginal or experimental use cases, thus increasing the potential for pervasive use of D&A across the organisation.
- Better understanding and sharing of resources and capabilities enables greater value to be created: The opportunity to reuse capabilities across the organisation not only offers more return on D&A investment but also allows deployment of common functionality across multiple different use cases (such as embedding common reporting functionality into multiple different operational systems).
- Integration of D&A into the organisation and societal ecosystem has a broad and diverse impact: Data and analytic governance needs to embrace discussions about how to reconcile a shortage of human skills with:
Increasing technological capabilities
Issues of Data Sovereignty
The acknowledgement of data privacy and security expectations
Considerations of the environmental impact associated with the sourcing of technology and power.
Trends reflecting this theme:
- Data sharing is essential is a necessary part of understanding the organisational ecosystem.
- D&A sustainability connects the organisation’s behaviour to its impact on the broader ecosystem.
- Observability traces the flow of data and insight throughout the ecosystem.
- A practical data fabric enables the construction of the ecosystem.
- Emerging AI will transform the ecosystem, especially by integrating humans in new ways.
- Converged and composable ecosystems are the new technological bedrock to systems delivery.
Theme 3: “Don’t Forget the Humans”
Concepts such as robotic process automation (RPA), techniques such as AI, and use cases such as personalised product selection, driverless cars and algorithmic trading are all designed with an underlying expectation that things would work much better (or at least more cheaply) without humans. However, several factors are driving a more balanced view of D&A that accepts that, although AI tends to outperform humans (in some narrow use cases), AI plus a human tends to deliver better results than AI alone. In certain use cases, it is even undesirable to remove humans from the process.
This focus on retaining the human dimension of D&A has three key implications:
- Driving adoption of D&A by working with business users to ensure accessibility, trust and relevance to business users: Giving workers the ability to control their environment has repeatedly proven to increase performance and satisfaction. Giving data and analytics users the power to influence or even control the way analysis is created and used increases the likelihood of its use in their decision-making.
- Recognising human involvement across the range of decision-making, from decision support to decision automation: Even the most automated decision-making process needs human involvement in its initial design and formation and its subsequent monitoring and evaluation.
- Ensuring D&A deployments consider multiple aspects of risk: The risk of rogue AIs, and humanity disenfranchised by ubiquitous technology or environmental collapse are science fiction tropes for a reason: All are catastrophically plausible. The need for D&A leaders to guard against the worst possible implications of trends in D&A while ensuring the best potential outcomes requires careful attention and diligent governance.
Trends reflecting this theme:
- Managing your AI risk is the recognition that AI delivers unpredictable impacts that require careful governance.
- D&A sustainability traces the impact of D&A on the human environment and quality of life.
- Emergent AI transforms the relationship between humans and organisational systems.
- Consumers becoming creators reflects the changing role of business users in modern D&A from being passive consumers of insights to actively engaged insight creators.
- Humans remain the decision-makers makers acknowledge the primacy of humans in the decision-making system.
Value Optimisation
Description:
Value optimisation from an organisation’s data, analytics and AI portfolio requires an integrated set of value engineering activities and competencies. First, building a value story identifies and articulates stakeholder impact on processes, activities and decisions from D&A initiatives in business terms. This is needed to get buy-in from business peers and establish how results will be measured.
This also provides the foundational value assumptions needed to prioritise investments as part of portfolio optimisation D&A strategy and operating model development. Value stream analysis and design thinking are techniques that can help identify potential high-value, cross-enterprise options and decisions. Measuring outcomes using outcome-driven metrics that link technical outcomes to business outcomes and adjusting the portfolio as needed ensures the organisation is realising the expected value.
Why Trending:
- Ongoing and constant business and technology disruption is putting increased pressure on all business and technology leaders to demonstrate the value of their initiatives.
- Most D&A leaders struggle to articulate the value they are creating for the organisation in business terms. A belief case (as opposed to a business case) is no longer enough. Only 35% of respondents were effectively meeting the objective of showing demonstrable and verifiable value to data and analytics stakeholders.
- D&A is increasingly distributed in business lines with significant benefits to business domains. However, alignment and collaboration with D&A teams are needed to ensure D&A-enabled change, organisational agility to respond to change and connected decision-making.
- Focusing solely on ROI to guide investment decisions leads to overweighting tactical versus disruptive benefits and misallocation of resources.
Implications:
- D&A leaders must show a clear link between D&A initiatives and the business outcome improvements to processes, activities and decisions tied to the organisation’s mission-critical priorities. Otherwise, data and analytics will be viewed as a cost centre ripe for reduction when resources are constrained rather than as a strategic growth and innovation driver.
- Discovering and aligning to value streams to deliver D&A initiatives maximizes desired business outcomes while efficiently utilising scarce resources and reducing excess system waste.
- Investments in data and analytics initiatives can yield short-term financial returns in revenue growth, productivity, and cost and risk reduction, but their more significant impact is often on leading indicators of financial outcomes and future value. These financial-influencing benefits (including an organisation’s brand value; know-how; innovation; organisational agility; achievement of mission; leverage to other projects; and employee and customer retention, acquisition and loyalty) are often excluded from value assessments. A comprehensive approach to value identification, communication and option selection is needed to optimize value realisation.
- Most D&A organisations build business cases to get funding and then deliver the technology but never measure the actual business impact and results. Measuring business outcomes is needed to understand the true value that data and analytics deliver.
Actions:
- Optimise value by building value stories that establish clear links between D&A initiatives, the organisation’s mission-critical priorities, and the stakeholder business outcomes and value they create. The value story should include outcome drive metrics to link technical outcomes to business outcomes and should identify when adjustment is needed.
- Identify how data and analytics improve specific processes, activities and decisions is a critical piece of identifying stakeholder business outcomes.
- Make the best possible resource allocation decisions by creating a consistent approach to scoring investment options across financial and financial-influencing value and risk. Mature organisations establish a value council consisting of D&A and business leaders to measure results and embed value engineers into product fusion teams.
- Maximize the business value of your D&A investment by exploiting value stream analysis to connect related decisions to maximize decision impact and minimize unintended consequences.
- Realize expected business outcomes and value by building a value stream management competency that combines value stream identification, embedded DevOps and lean thinking with data and analytics delivery processes.
- Drive decentralised D&A users to work together to speed up the iterative development of D&A solutions by establishing cross-functional fusion teams supporting your value streams. Enhance these cross-function, cross-discipline teams with lean and agile practices.
- Early adoption of new technologies. It is a given that there are millions of specialist development companies around the world offering far more advanced solutions than you might yet have thought of. Often created as a solution to someone else’s concerns, chances are that they would be a tremendous boon to your efforts, and worth perpetual investigation and awareness.