Real-time analytics getting it right. As data of all types pours into enterprises at unprecedented rates, being able to give decision-makers easy access to immediate insights is becoming a business necessity. Real-time analytics enables organisations to meet this challenge by applying logic and mathematics to raw data, transforming numbers into actionable knowledge, and opening the door to rapid and accurate decision-making.
As with any powerful IT tool, real-time analytics requires a high degree of understanding and proficiency before it can be used to meet critical real-world business needs, such as enhancing workflows, boosting marketing and sales programs, and understanding various types of customer behavior.
Here are critical ways an organisation getting started with real-time analytics can avoid common mistakes and drive the technology toward its maximum potential.
Limit real-time analytics to relevant use cases
Business and IT leaders are often so enamored with speed that they insist every data insight be delivered in real-time. In some situations, delivering real-time analytics is not only a waste of money but also counterproductive.
For example, putting your financial revenue report in a real-time analytics environment may not be appropriate, especially if orders are frequently cancelled, moved, or manipulated. How would the sales manager react when one minute she’s hit her target and the next, in real time, she’s dropped to 88%?
By understanding which analytics can truly benefit from real-time support, IT can ensure real-time analytics initiatives generate significant value for the enterprise. Like any analytics effort, you need to have a strategy for what the analytics will produce and what decisions will be made from the analyses.
Build a strong and reliable infrastructure
Disappointment can occur when an enterprise, anticipating accurate real-time insights, fails to achieve these goals simply because its infrastructure doesn’t live up to planned performance levels.
To ensure long-term success with real-time analytics, the underlying architecture needs to support real-time data manipulation, ingestion, and processing. Models also need to be built to support the processing of real-time data, and the data sources must truly be real-time, not near-real-time or daily-generated batches.
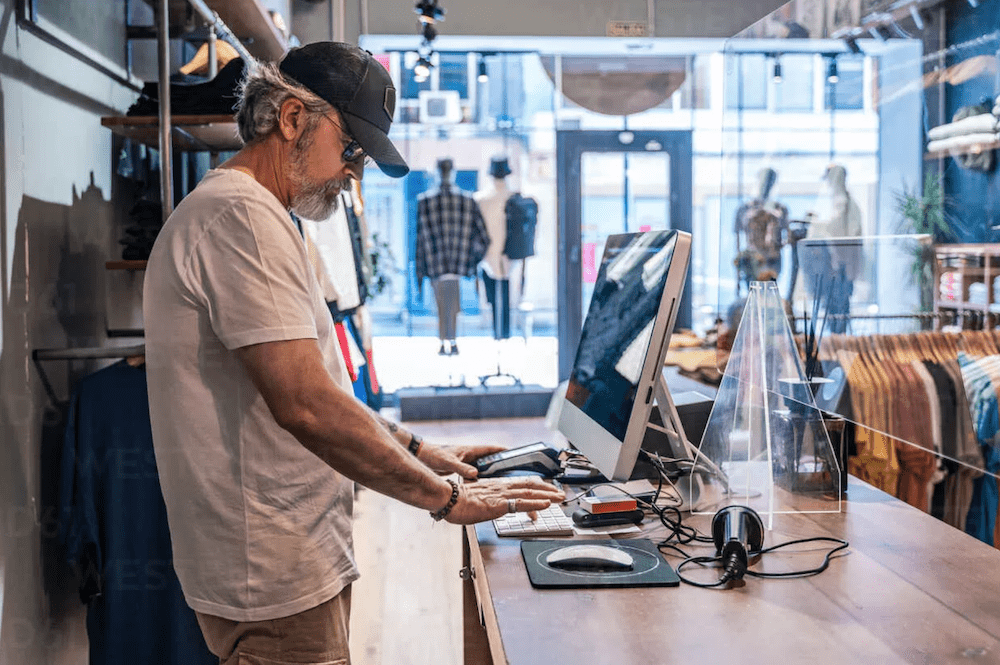
Deploy applicable dashboards
Before moving forward with any real-time analytics initiative, the project leader should reach out to end users to identify the types of dashboards they need. Armed with that information, the IT leader can have his or her team review the data capture requirements from the source data and ensure that the real-time analytics solution can provide the information the end-user needs in exactly the formats they need to see them in.
Temple explains that the approach allows IT to place the end user at the center of the analytics discussion. Rather than foist an analytics solution that can disrupt a user’s workflow, doing the leg work to identify needs and offer insights into what’s possible before attempting to buy and deploy an analytics system will result in analytics that are uniquely targeted to individual user needs.
Combine real-time data with historic data
Real-time data’s value increases exponentially when it’s merged with historic data, enabling end users to combine and compare insights in the moment.
Consider, for example, temperature data that’s transmitted by a sensor embedded inside a machine. Understanding such data in real time is useful for checking that the machine is operating efficiently or that a temperature threshold hasn’t been reached. When historic data is mapped over days or weeks, decision-makers can gain a richer understanding of how a particular machine is performing. You can also build predictive models based on other machine performance profiles.
Incorporate both internal and contextual data
IT leaders need to ensure that their analytics practice can ingest not only internal data but also contextual data related to competition, markets, customer segments, and census data points to provide a comprehensive set of facts and trends. There should also be a direct input into the organisation’s business technology roadmap and long-range financial plan.
Over weeks and months, access to real-time internal and contextual data, rich with meaningful insights, can transform an organisation’s decision-making process. This approach is effective because it focuses on transforming the culture of an organisation by becoming more data-driven, fact-based, and macro-aware of their business landscape.
Focus on delivering material information
A concept borrowed from law and accounting, “material information” is information that causes a recipient to change their thinking on a particular matter or alter their judgment or course of action. They will do something in response to receiving the material information.
For example, a vehicle’s oil gauge may only be checked once, if at all, during the course of a journey. That’s static information. But if an alarm illuminates or sounds, the driver will immediately consider seeking a solution at the very next exit.
Executives and managers are flooded with information — far too much to ever absorb completely. However, only … a very small amount of material information needs to be analysed in real-time to ensure success.
Build an analytics team that understands key business needs
The analytics team should be a true partner, not simply an order taker. When the analytics team is seen as a business enabler and not a cost center, the enterprise will be willing to invest more in people resources and technology to support the analytics function.
The best way to ensure long-term success is to furnish the analytics team with business knowledge so it can provide relevant intelligence. The more they know about the business, the better they will be at delivering analytics that matter.
Over time, a well-supported analytics team will gain the ability to provide increasingly relevant data that enables decision-makers to take fast, well-informed action. This includes bringing in market data through API connections and data scraping, to support internally generated analytics. Being a partner through real-time analytics and telling your executive team things they wouldn’t know on their own, or even think to ask, will make you a rock star.