Propensity models, also called likelihood-to-buy or response models, are what most people think about with predictive analytics. These models help predict the likelihood of a certain type of customer purchasing behaviour, like whether a customer who is browsing your website is likely to buy something. This helps marketers optimise anything from email send frequency to sales staff time, to money, including discounts.
An example of a company using predictive analytics using the propensity model is online pet products company Fin & Fur has served pet owners for more than 20 years. It sells many products that customers have to re-order at varying times from three months to 12 months. Like most retailers, Fin & Fur had previously taken a one-size-fits-all approach, offering a set calendar of products at set times and promotions to all customers. But not all customers are alike and many are looking to buy different products at times, to say nothing of having different pets!
Using predictive personalisation software, Fin & Fur were able to differentiate products across customers, leading to higher sales and retention without increasing costs. Customer differentiation was incorporated according to their highest likely product choice to purchase.
Based on that ranking, they were able to determine which products would obtain the optimal response from each customer and maximise the capture of those returns using an automatic email feature. This surgical precision drove incremental margin from customers who were already motivated to buy and incremental revenue from customers who had hitherto felt no incentive to buy.
Thanks to this and other predictive marketing campaigns, quarterly sales increased by 38 per cent from the year before, profit rose by 24 per cent, and customer retention increased by 14 per cent. Plus, the changes allowed Fin & Fur to more than double its campaign response rates without increasing the marketing or promotional budget by a single dollar.
Propensity models likelihood – response models predictive analytics
To predict which prospects are ready to make their first purchase, a likelihood-to-buy model evaluates non-transaction customer data, such as how many times a customer clicks on an email or how the customer interacts with your website.
These models can also take into account certain demographic data. For example, in consumer marketing, they may compare gender, age, and zip code to other likely buyers. In business marketing, relevant demographics may include industry, job title, and geography. Predictive personalisation, working autonomously, automatically appreciates the uniqueness of the visiting customer activity, capturing every impression made as they navigate through the website.
Here’s how it works: the model watched each individual as a stand-alone entity. No reference to “people who bought that also bought this” is made, as it is irrelevant to your consumer. However what is significant and of utmost importance is what actions that individual takes when resulting in purchase.
If they check something out 3 times, only purchase on a Tuesday, only purchase if they look at something 5 times etc, all unique to that individual alone. Exactly like a “tell” in poker. It perpetuates throughout their lifetime and the algorithm learns that an individual’s behaviour pattern, indeed matures with them perpetually. Once you’re armed with this data, you have a heightened likelihood of a successful return from each prospective customer.
Predicting the Likelihood to Buy for First-Time Buyers
For consumer marketers, likelihood-to-buy predictions allow you to decide how much of a discount you might allocate to a certain customer because people who are already more likely to buy won’t need as aggressive of a discount as customers who are less likely to buy. The models then get better over time, as companies collect more data and automatically test whether predictions become reality.
For instance, the large European household appliance manufacturer Arcelik maintains a call centre where employees are given a list of customers who are likely to be ready to buy a new washing machine within the next few months. Agents then make calls to these customers with offers such as a year of free detergent with the purchase of a washing machine. The tactic works well for considered purchases, such as refrigerators or cars, and larger-ticket items such as high-end fashion apparel.
A high-end shoe brand provides store associates with lists of customers to call to. The store associates have already developed strong relationships with their customers, but they can be even more successful when armed with predictive analytics. Employees can now see which customers are likely to be interested in a certain style when a new season’s shoe comes out, based on customers’ past behaviour or how similar their purchase habits are to other customers.
Employees can then reach out to customers with that information. A call could go something like this: “Hi Joe, it has been a while since we’ve spoken. I just wanted to let you know that there is a new cross-country running shoe I think you might like. It’s similar to the shoes you bought two years ago but in a new material. I have put a pair aside for you in your size. If you have time, perhaps you could stop by on your way home from work to have a look?” Who would not want to receive a call or an email like that from their shopper?
As reported by the New York Times and others, President Barack Obama used propensity models, specifically propensity to vote for the Democratic Party, to help him win reelection in 2012. His staff of volunteers could not possibly meet with every voter in the country so the challenge was to find the undecided voters.
There was no point spending time or money trying to woo die-hard Republicans who would not change their minds anyway, or die-hard Democrats who were already likely to vote for Obama. Rather, using propensity models, Obama’s team of data scientists found those voters who were undecided but could still be persuaded. They then focused on finding already strong Obama supporters in the undecided voter’s social circle and asked them to spend time with the undecided voter to explain their views.
Predicting Likelihood to Buy for Repeat Buyers
What good is spending money to acquire new customers if they only buy once and do not return? Based on a customer’s propensity to purchase, it is not only important to predict the likelihood to buy for first-time buyers, but it is equally important to predict the likelihood to buy for repeat buyers. Your goal is to keep customers coming back time and time again. It is happy and loyal customers who have a large lifetime value, and many customers with a large lifetime value make for large revenues and profits for your company.
Predicting the likelihood of buying for repeat buyers is a lot easier than predicting the likelihood of buying for first-time buyers because there is a lot more information to go on. The likelihood to buy model for repeat purchases evaluates earlier transactions as well as other interactions similar to the model for prospects.
However, the added information derived from the first purchase can significantly improve the accuracy of the likelihood-to-buy model for repeat purchases, as compared to a similar model for prospects. Unlike the first purchase predictions, repeat purchase predictions utilise all interactions of the customer, such as past purchases, returned purchases, and phone calls to customer service.
Choosing the Right Level of Discount Using Likelihood to Buy
There are two main uses for likelihood-to-buy predictions: which customers to focus on and how much money, including discounts, to spend on each customer.
Choosing your audience carefully is important if you want to optimize marketing ROI, because reaching customers can be expensive. For instance, a direct mail or call campaign that costs $1 per customer interaction and has a 2 % purchase rate could cost $50 a person before a discount is even given.
If you can target your audience and make the communications more relevant, the purchase rate could be significantly higher, say 10 %, reducing the cost to reach each buyer significantly. In addition to choosing the right people, you can increase purchase rates by including relevant recommendations or content and to communicate with customers at the right time. Focusing on relevancy will allow companies to reduce their dependency on high discounts. Using this strategy, it is possible to significantly reduce discounts as a part of their customer acquisition strategy.
Discounts and other incentives can still be used as a sweetener in necessary cases, such as targeting a customer who has abandoned her cart. However, we recommend you do not give out discounts to everybody or else you will train your customers to get used to discounts.
Instead, start with recommendations and reminders and use discounts only if you need them. The lack of understanding of the individual customer leads to blanket discounts, which reduces overall profit margins considerably. Only about 20 % of customers are “discount junkies,” people who will only make purchases when given a discount, according to an analysis of 150 retailers.
About 15 % generally pay full price for most of their products, while the majority of customers fall somewhere in between, the data show. By analysing their customers’ behaviour, marketers can determine which customers may need more encouragement in the form of a gift or discount. It can also help marketers flag customers who will come back and buy anyway, so no additional monetary encouragement is needed. This model helps to maximize both revenue and profitability from each member of your customer base.
Targeting discounts is good for business and good for customers. By surgically targeting discounts, retailers avoid margin erosion, which in turn reduces the price increases retailers would normally have to take to make up for the lower profit margins. That practice effectively lowers prices for all customers. This is a simple but very powerful paradigm shift for marketers who traditionally focus on product lines, merchandising, and one-size-fits-all discounting. Behavioural and lifecycle data available at the customer level combined with the predictive insight about the likelihood to buy allows marketers to surgically discount to maximise margin and customer lifetime value.
Also, if you are going to include an incentive or discount to acquire, retain, or reactivate a customer, there is no need to go beyond the level of discount that customers are used to seeing. Predictive analytics allows you to tailor offers based on what level of discount triggered past customer purchases. Customers with a high likelihood to buy may get a lower discount but perhaps other perks such as early access to products to drive more purchases. Customers with a low likelihood to buy or those who only buy on discount (a behaviour-based cluster) might get a higher discount.
A multichannel retailer of sports goods used to send all of its customers a 50 % clearance discount at set times during the year. This campaign was motivated by its desire to clear old inventory. In other words, it was a product or merchandising-centric campaign, not a customer-centric campaign.
This campaign trained customers to wait for the big annual sale and brought in a few dollars for the retailer. Using predictive analytics, this company analysed the likelihood of buying and discounted the sensitivity of all of its customers. If a customer is likely to buy with an offer of 25 % off, you don’t need to give them a 50 % discount.
Using predictive analytics, the retailer identified the right discount level for different groups of customers. Now the retailer still sends discount emails at set times, but it will send different levels of discounts to different groups of customers. It sends just enough to get the customer to buy, but not too much to give away unnecessary margin. Using this approach, the retailer was able to achieve an overall increase in revenues of 20 %.
Predictive Lead Scoring for Business Marketers
If you are in business marketing you can equally prioritise your investment using likelihood to buy models. You can ensure that your sales team spends most of their time with the prospects that have the highest likelihood of becoming buyers. This can have an enormous impact.
Consider the following example in marketing business software. Let’s say you have a free trial for your software. Not all people who sign up for your free service have a serious intent to buy. It is not uncommon that 70 % of free-trial signups are made just out of curiosity without an immediate need or budget to buy.
Another 20 % are serious evaluators and 10 % are on the fence: they could go either way. If you randomly call people from a list, perhaps based on company size, it is easy to see how you may end up wasting your entire day on prospects that aren’t serious. It is especially important to prioritise your time if you are serving small- and medium-sized businesses, of which there may be millions.
The main difference between predicting the likelihood to buy in consumer and business marketing is due to the nature of the purchase decision process. In most business marketing, the decision process is long and complex.
With a considered purchase, which most business marketing falls under, the decision process is longer and includes many interactions between the marketer and the buyer. This requires special attention to focus on all prospects within the decision funnel. Hence business marketers turn to all interactions and signals from prospects to determine who is most likely to buy and therefore worthy of time and attention.
The below gives an overview of lead scoring methods beyond predictive methods.
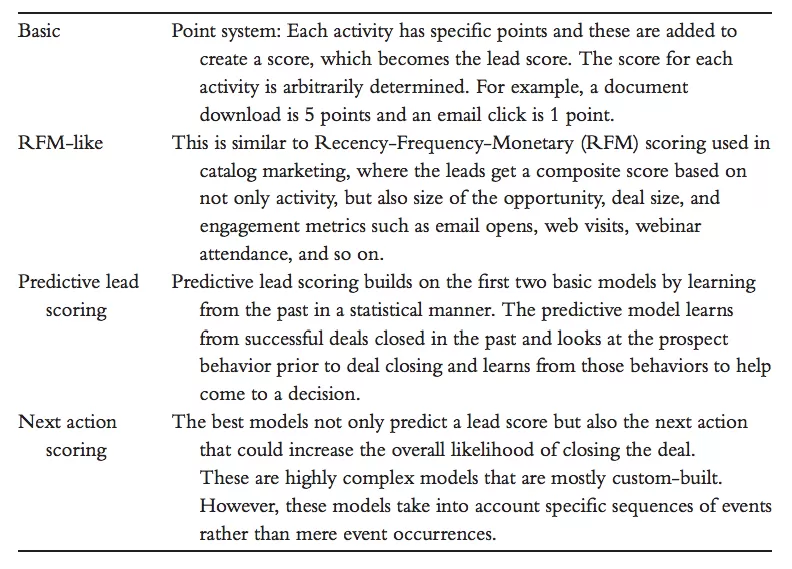
Because the replacement and delivery cycles for vendors, deals, services, and products can take a long time, most B2B marketers are hyperfocused on acquiring new customers, rather than getting existing customers to come back, where likelihood-to-buy first purchase models take greater importance.
Predictive models are not the only way to prioritise prospects for business marketers. However, predictive models are by far the most accurate and relatively easy to use.