Do you ever get the feeling that where you think your income is coming from might be wrong? Installing new marketing software to improve ROI can be fraught with danger. There is even a nightmare scenario causing you to refuse to believe an incumbent might have claimed attribution for returns outside possibility. Human nature won’t let you believe figures that, having been relied upon for several years, have been wrong.
The increasing complexity of the customer journey, a broader marketing landscape, and the need to justify marketing spend and ROI are driving more and more marketers to demand better attribution of their marketing activities.
Much of the discussion centres around why attribution matters and the selection of a particular attribution model. Both of which are important. However, for us, a more pressing issue is the flaws in these existing models. And, just as importantly, the poor quality of data that is being fed into them is hampering their accuracy and effectiveness.
Existing flawed attribution models are part of the problem
At the most fundamental level, almost all current attribution models are historical and all the evidence is that they are woefully inadequate in terms of doing what they should.
For example, Last Click is the default attribution model for GA. It is a complete oversimplification, where 100% of the value of the conversion is given to the last marketing engagement. In essence, this ignores all the previous points in the buyer journey: almost the same as applying no attribution at all.
Google 360 also has limitations in terms of a short lookback window and only partial attribution about what is very often a long and complex customer journey. So, rethinking today’s attribution problem and rebuilding attribution – in a way that reflects the complexity of the customer journey – has to be a key goal for all marketers.
Unfortunately, “in the wild” the vast majority of marketers – in fact over 90% of those responsible for improving marketing ROI in the UK – are unable to get to sophisticated attribution models that are fit for purpose or even simple rules-based models like Time Decay-Weighted or Position based
Your core analytics data is likely 80% wrong
Extensive work around data enrichment also suggests that flaws in existing attribution models are only one part of the story.
Just as important is the quality of the underlying data that you are feeding into your attribution model – and in purely layman’s terms if you put rubbish in, you will simply get rubbish out. Typically, we find that marketers are struggling with key issues around the data they are feeding into their attribution models:
Limitations in the cookie-pixel approach
Google’s approach to collecting the vast majority of digital marketing data is based on a device accessing a web property. Not an individual who will use multiple devices in the course of even a simple transaction.
As a result, it does a poor job of “joining” multiple sessions and generates data that is around 80% incorrect. This is based on a real analysis of attribution at one of the UK’s largest retailers where Google 360’s approach was compared to something more accurate.
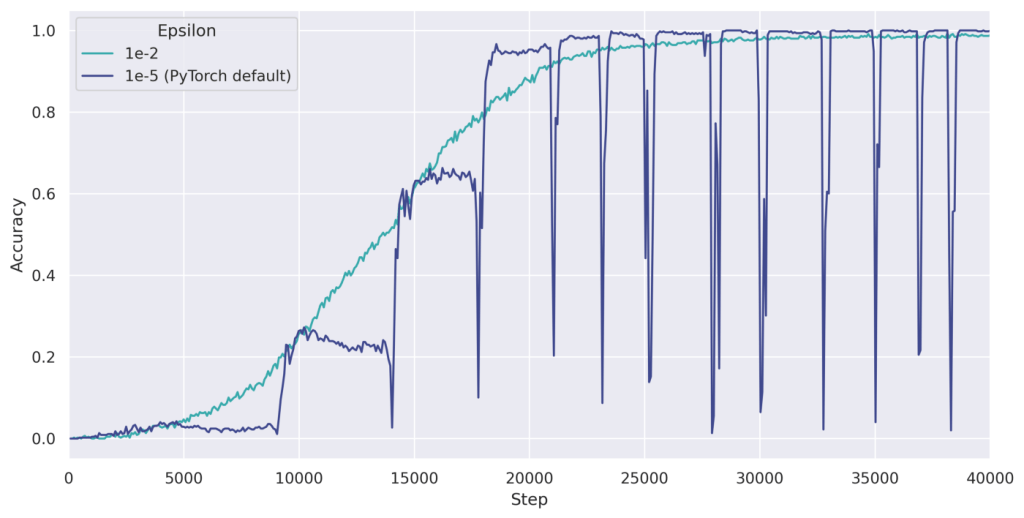
Challenges with integrating offline data
Offline increasingly offers rich data as measurement moves beyond traditional panel-based approaches to incorporate Beacon technology, opt-in Wi-Fi Tracking, and RFID data.
But there are real challenges with integrating this type of econometrics data including the inability to look beyond channel-level impact, a lack of immediacy in terms of delivering data outcomes, and the fact that they operate in a silo to all other data collection strategies. And they can be expensive.
Challenges in mobile application & social data collection
Data collection in-app or by a social platform can create a much more robust measurement environment. However, the challenge today with activating mobile app data is building good-quality data to connect it to.
It also has limitations in the way that significant volumes of retargeting activity occur against social platform pixels – essentially short-cutting the need to understand the customer journey. There’s also the broader issue of “walled garden” approaches being taken by major social platforms
Fingerprinting & 1st party enrichment of data
Device-led and Canvas Fingerprinting offer the opportunity to combine certain attributes of a device to uniquely identify it and ultimately the user. However, current limitations – for example, the fact that this approach is blocked by default for Safari users – and changes to 3rd party cookies are increasingly making this approach ineffective.
So, if there are issues around not only the attribution models you are using – but also the underlying data you are feeding into them – where do you go from here?
Why rebuilding attribution is the key
It is possible to move past some of the limitations that were identified earlier in single or multi-touch attribution approaches – by using statistical or data-driven models, Media MIX Modelling (MMM)/Econometrics or even Probabilistic modelling. And each of these has its own merits.
But, ultimately, they also all share some of the data quality challenges identified above and are restricted in terms of the views they can provide – for example, being limited by period or specific channel.
Crucially, their respective views of the customer journey are truncated and they aren’t able to provide an understanding of the impact that a specific marketing touchpoint has on a customer’s own, very unique journey.
It is these very limitations that have led to the development of a new approach to marketing attribution – visit-level attribution.
A completely new approach: Visit level attribution
Advances in technology have made this a reality.
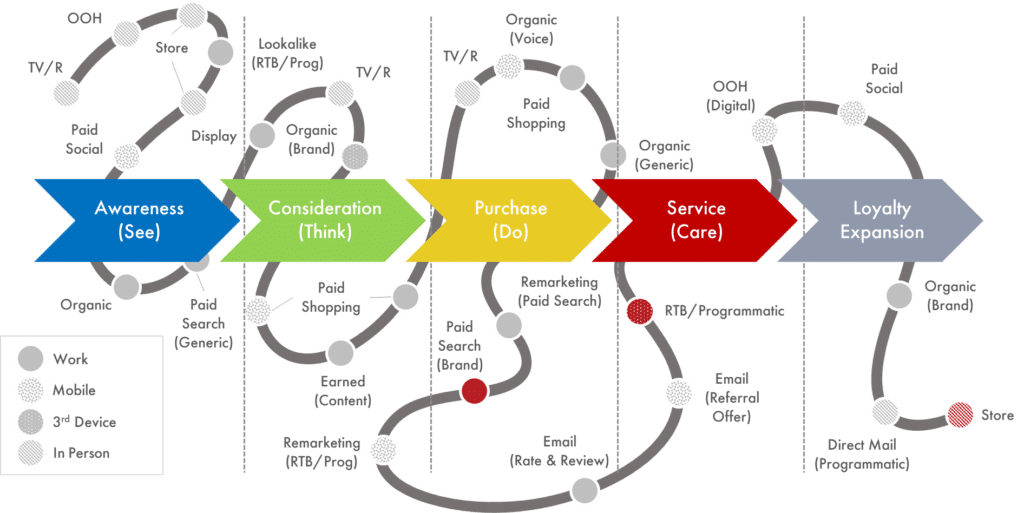
It is now possible to take an approach that learns from econometric modelling techniques but is focused on providing near-live data for tactical marketing insight using bleeding-edge data science. This leverages Machine Learning techniques to rebuild your marketing data to identify visit-level attribution and create a fully attributed map of a customer journey.
Consider the impact of a piece of content on one particular prospective customer journey. It enables you to unravel the complexity of the wide variety of touchpoints on individual customer journeys and accurately attribute the correct level of impact to each.
This allows you to make the direct link between marketing spend and revenue, providing the capability to significantly improve your marketing ROI by making informed judgements based on sound data analytics. The true goal of marketing attribution.
Talk to us
If you’re currently facing issues with your ecommerce marketing, hyper-personalisation or attribution, talk to us about how we can make SwiftERM work for you.