Large Language Models (LLMs) are neural networks with multiple parameters. They are trained on large volumes of text data through semi-supervised learning. The most well-known example of an LLM is ChatGPT.
LLMs and data analytics work hand in hand to provide valuable insights. This connection turns them into essential tools for data leaders and professionals in various industries. As LLMs become more and more complex, they continue supporting a wide variety of generative AI use cases that drive businesses forward.
Understanding LLMs: The Basics
Large Language Models are advanced artificial intelligence systems that excel at processing and generating human-like text. These models employ deep learning techniques and leverage extensive datasets to comprehend and generate natural language.
By training on massive amounts of data, LLMs develop an understanding of patterns, grammar, and context necessary for generating coherent and contextually relevant text. They possess the capability to:
- Comprehend prompts
- Engage in conversations
- Summarise information
- Produce creative pieces of writing
- Analyse text, video, and audio conversations
Over the years, LLMs have undergone significant changes. Beginning with early language models like Eliza and ChatGPT, advancements in machine learning and neural networks have led to developing more sophisticated models such as GPT-4 (Generative Pre-trained Transformer 4). These models have grown in size and complexity, enabling them to handle a broader range of tasks and generate higher-quality text.
A potential concern is the expense of using LLMs, particularly their cloud computing and sustainability impact:
However, as LLMs become more refined and targeted to specific business challenges, their computing and power requirements will also diminish, making them more practical for various Gen AI use cases at companies of different sizes.
What is the difference between GPT and LLM?
GPT (Generative Pre-trained Transformer) models are specifically designed for text generation, text completion, and various language-related tasks. LLMs (Large Language Models) are a broader category that includes different architectures such as Transformers, RNNs (Recurrent Neural Networks), and CNNs (Convolutional Neural Networks).
LLMs are designed to be scalable and versatile, with the choice of architecture depending on the specific objectives of the model. While GPT models are focused on language tasks, LLMs encompass a more comprehensive range of applications, including data analytics and other non-language tasks.
The Importance of LLMs in AI
LLMs are crucial in advancing AI capabilities, particularly in natural language processing (NLP). They can potentially revolutionise various industries, including customer service, content creation, language translation, and more.
These models can automate tasks, provide individualisation or hyper-personalised recommendations, and generate human-like product selection choices unique for each consumer. Their ability to comprehend and interpret vast amounts of textual data opens up new possibilities for leveraging AI in various applications.
Understanding Data Analytics: The Basics
Data analytics refers to the process of examining and interpreting large volumes of data to uncover meaningful insights, patterns, and trends. It involves applying statistical and analytical techniques to extract valuable information from raw data.
Data analytics aims to gain a deeper understanding of the data and use that knowledge to make informed decisions or drive improvements in various aspects of business operations.sArtificial intelligence and data analytics are related but distinct concepts. While AI involves the development of intelligent machines capable of performing tasks that typically require human intelligence, data analytics focuses on analysing historical data to extract insights and drive decision-making.
Data analytics uses statistical techniques and algorithms to uncover patterns and trends within the data, enabling ecommerce organisations to understand past performance and make data-driven decisions.
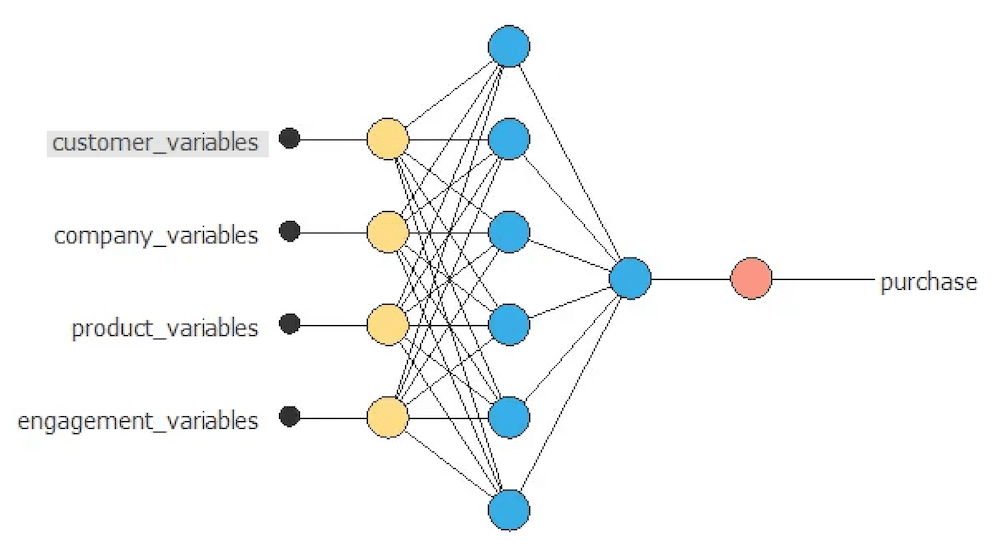
Data analytics is vital in modern businesses, impacting decision-making processes across various industries. Here are a few reasons why data analytics is crucial:
Improved Decision-Making
By analysing historical data, organisations can identify patterns and trends unique to each customer far beyond that person’s buying history alone, enabling them to make informed decisions based on evidence rather than intuition or guesswork. Data analytics provides valuable insights that can guide strategic planning, optimize operations, and enhance overall business performance.
Enhanced Efficiency and Productivity
Data analytics allows businesses to identify inefficiencies, bottlenecks, and areas for improvement. Uncovering hidden insights within the data allows you to streamline processes, allocate resources more effectively, and improve operational efficiency.
Hyper-personalisation
Data analytics helps businesses better understand their customers by analysing their behaviour, preferences, and purchasing patterns. This knowledge enables you to deliver personalised experiences, relevant product offerings. This results in increased customer satisfaction and loyalty.
Competitive Advantage
With data playing a significant role in successful business operations, organisations harnessing data analytics’ power gain a competitive edge. By leveraging data insights, businesses can identify market trends, predict customer needs, and stay so far ahead of the competition that it defies belief.
LLMs and Data Analytics, achieving individualisation – the Intersection
The combination of LLMs and data analytics brings together the power of advanced language processing and the ability to extract insights from large volumes of data. LLMs can be utilised to analyse textual data, such as customer reviews, social media posts, or survey responses, and extract valuable information.
By applying natural language processing techniques, LLMs can run sentiment analysis, identify key topics, and extract relevant keywords.
Modern LLMs have evolved to process and generate text and perform data analysis tasks. They can assist in data preprocessing, such as cleaning and organising data, and even create visualizations to present data in a more digestible format. LLMs can identify trends, correlations, and outliers within datasets, cognitive dissonance for example, enabling businesses to gain valuable insights and make data-driven decisions.
By incorporating LLMs into data analytics workflows, businesses can gain a more holistic view of their data, bridging the gap between structured and unstructured information. This integration allows for a deeper understanding of customer sentiments, market trends, and emerging patterns that might otherwise be missed.
By implementing LLMs into data analytics processes, businesses can unlock the potential of unstructured data, enabling them to make more informed decisions.
Overall, this tandem usage boosts business intelligence by leveraging the power of language processing and data analytics to drive strategic decision-making and foster growth.
The Power of LLMs in Predictive Analytics
Predictive analytics is a branch of data analytics that utilises historical data, statistical algorithms, and machine-learning techniques to forecast future trends, behaviors, or outcomes.
By analysing patterns and relationships within the data, predictive analysis aims to make accurate predictions and inform decision-making processes. It enables businesses to:
- Anticipate customer behaviour
- Identify potential risks
- Optimise operations
- Gain a competitive edge
LLMs can play a significant role in predictive analysis by leveraging their language processing capabilities. While LLMs are not specifically designed for numeric data analysis, they excel in processing and generating textual information.
They can analyse large volumes of text data to extract insights that can be used in predictive modeling. LLMs can assist in identifying relevant features, sentiment analysis, and understanding the context of textual data, which can enhance the accuracy and depth of predictive analysis.
The integration of LLMs in predictive analysis offers several benefits for data-driven businesses:
Enhanced Contextual Understanding
LLMs can provide a deeper contextual understanding of textual data, enabling more accurate predictions. They can identify sentiment, detect nuances, and interpret the semantics of written content. This, in turn, can improve the accuracy of predictive models.
Uncovering Hidden Insights
LLMs can uncover hidden patterns, trends, and relationships within textual data that may not be apparent through traditional data analysis techniques. By incorporating LLM-generated insights into predictive models, businesses can gain a competitive advantage and make more informed decisions.
Enriched Feature Extraction
LLMs can assist in feature extraction from textual data, which can enhance the predictive power of models. By identifying relevant keywords, topics, or themes, LLMs contribute to the creation of more robust predictive models.
While they offer significant advantages in processing textual data, LLMs have limitations when it comes to creating predictive models using tabular (numerical) data. LLMs are primarily trained on textual data and may not be suited to handle numerical data or structured datasets.
As a result, they may not reliably generate accurate predictions when working with numerical data. To address this limitation, businesses may need to combine LLM-generated insights with other predictive analytics techniques tailored explicitly for numerical data analysis.
LLMs and data analytics are vital tools in today’s data-driven world. Their intersection has led to enhanced business intelligence and improved predictive analysis.