Content marketing focuses on creating and distributing useful, pertinent material to engage with your target market. The goal is to grab their interest maintain it and establish a durable bond. That intangible might be more easily identified as perpetually relevant to each individual’s needs. A successful content marketing approach is a powerful amplifier for your brand in the bustling market. It’s not merely about making sales; it’s about leaving a memorable mark that echoes when customers are in the decision-making phase.
Ultimately, it’s about narrating your story in a manner that remains memorable and ignites that connection with your audience.
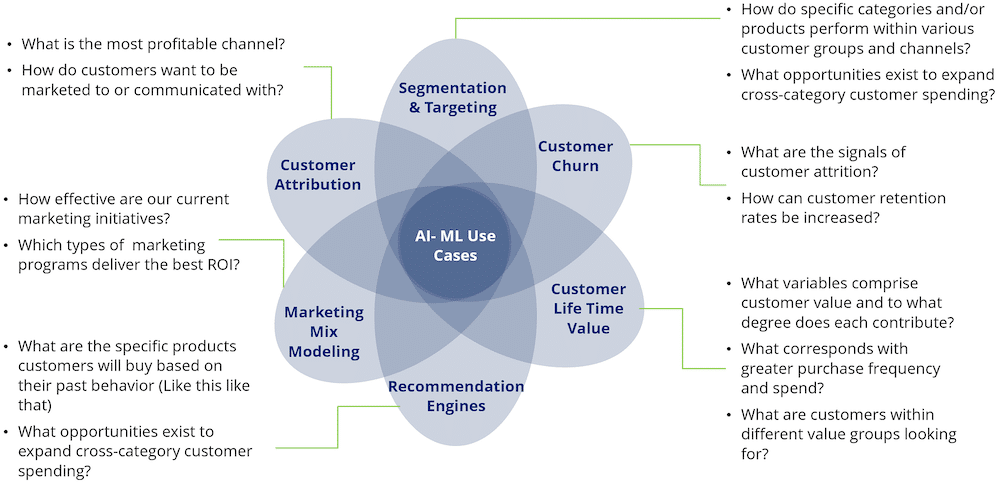
Marketing attribution, and especially sustaining email attribution, serves as an ideal arena for data science, capable of integrating extensive data from diverse origins to assist marketers in comprehending the insights in a scalable manner, down to the most detailed level regarding the most effective (and least effective) sources of conversions. Following this, marketers can modify their budget allocations (either manually or through automated processes) as needed.
This perpetual drive for an improved method to tackle one of the most significant obstacles in marketing has transformed what was once a business inquiry into a data science challenge, fundamentally altering the fundamental question. Previously, the question was, “How can I increase the number of purchases of my product through advertising?” Now, it’s “How can I assess the impact of an advertisement on a customer’s decision to purchase?” or “How can we evaluate the success of each advertising platform?”
A deep dive into marketing attribution
It’s important to mention that the phrase “marketing attribution” frequently refers to three separate but distinct processes: Assigning offline results (like purchases from physical stores) to a specific campaign. Monitoring campaigns across various devices or media platforms (e.g., determining that a certain user initially saw an ad on TV, then went to the website via their phone, and finally completed the purchase on a tablet).
Evaluating the success of various tactics within a digital-focused campaign on a particular device (e.g., one user on a specific device views three different ad types, with which one was the most successful?). All three aspects of marketing attribution are intricate (and are becoming increasingly complex as customer experiences become more scattered across different platforms and devices), yet the methodologies for each are unique.
Traditionally, attributing marketing success has been a painstakingly manual task that frequently proves to be more challenging (and less successful) than hoped for. Regrettably, because they are relatively straightforward, many marketing departments opt for single-source or single-touch attribution, along with other heuristic models. These models rely on basic principles, such as linking desired results to a single touchpoint in the customer’s journey or giving equal credit to all channels throughout the journey.
Typically, heuristic models for marketing attribution oversimplify the process and often lead to inaccuracies, particularly for products or services with lengthy sales cycles and numerous interactions, as it’s common for multiple messages to contribute to the desired outcome. Heuristic models also bring about significant bias; for instance, models that focus on the first or last click may over-emphasise retargeting or Google search as the best platforms for ad targeting. So, if heuristic models don’t work well, what does?
This article doesn’t cover every single method or model, but the most effective and popular choices that data scientists have experimented with on real customers:
Markov Chain Modeling
The result of a Markov model is the likelihood that a customer will progress from one stage of their buying process to the next. In essence, it simulates the customer’s buying path, and from this simulation, it enables marketers to address the query: “What impact would the absence of channel X in my marketing approach have on the likelihood of making a sale?” This will ultimately yield a “subtraction impact” for each channel, allowing marketing departments to identify which channels hold the highest significance.
Game Theory and Shapley Value
By applying game theory to the analysis of marketing attribution, it’s possible to represent the relationship between customer interactions with marketing channels as a collaborative game, where each channel acts as a participant in this game, and collectively, all channels work together to achieve conversions. In simpler terms, game theory in the context of marketing attribution determines the credit given to each interaction for a conversion based on its contribution.
The Shapley value principle suggests that if two channels are interchangeable, they should receive equal rewards (in this scenario, credit for a conversion). Conversely, if a channel does not contribute anything to any combination of actions in a user’s journey (in marketing attribution, the various steps a user takes), that channel should receive the credit for the conversions it generates on its own.
How to Execute a Marketing Attribution Project
If you’re already acquainted with the basic seven steps involved in creating a data project, then you’re already equipped with the foundational knowledge needed to begin leveraging machine learning for the advantage of your marketing department. However, it’s important to keep in mind a few specific aspects when dealing with marketing attribution.
1. Understand the Business
In any data science initiative related to marketing, it’s crucial to start from the business perspective. Before exploring the data, the group should pause to address the following inquiries, ideally involving members from the business, marketing, and data teams collaboratively:
How many different types of campaigns do we have, and what is the desired action for each campaign For certain companies or specific marketing efforts, the aim could be to encourage a sale. For others, it could be focused more on increasing awareness, meaning that just a prospective buyer coming to the site would be seen as the target outcome. Regardless, the specific outcome or objective for every marketing initiative needs to be established, and it should be precise. The effectiveness of various attribution models could vary with different campaigns, making it essential to outline this before initiating any action.
What is the ideal way to deliver results that will have real business impact? Simply put, what is the end product? Is it a dashboard or the allocation of campaign funds in real-time, automated mode? Not establishing the end products before starting a marketing attribution project lays the groundwork for failure, particularly when there’s a lack of alignment between data scientists and marketing teams, leading to a product that the marketing team cannot utilise.
2. Get Your Data
Developing an effective data science solution for a business inquiry begins with accurately identifying the business requirements, but that’s just the beginning. The next crucial element is high-quality data. The initial phase involves outlining all the pathways and interactions a customer goes through to ensure no aspect is overlooked.
Following that, it’s vital to track every action a user takes across each relevant platform. However, it goes beyond just tracking. It’s also about comprehending the specific data associated with each interaction, its origin, and any potential constraints (such as missing information). Grasping the concept of attribution data is key not just for the precision of the models but also for gaining the confidence of the business teams and leaders in the results provided by the models.
3. Prepare Data
Once all the appropriate data sources have been identified, regardless of the algorithm selected for attribution, the subsequent step in every scenario is to verify that the data is clean and properly formatted. This involves creating and clearly defining user sessions. At this stage, it’s possible to identify gaps in the data, such as missing tags for specific channels.
If the process reveals any gaps in the data (like missing tags for certain channels, for instance), the most effective strategy is to halt and tackle the issue. It’s impossible to construct an accurate attribution model with incomplete data, so dedicating time to resolve the problem and ensure the data is correctly attributed before proceeding is essential.
4. Explore, Clean, and Enrich Data
At this stage, it’s essential to establish the framework for the project, as the rest of the data analysis process hinges on the chosen model for attribution. In the realm of marketing attribution, experimenting with various strategies simultaneously is neither feasible nor advisable (although, if you’re not currently employing marketing attribution, adopting a basic heuristic model could be beneficial to gain an initial understanding of the customer journey).
Different from other machine learning models, such as churn prediction, predictive maintenance, or detecting anomalies, where it’s feasible to divide the data into training and testing sets to evaluate the model’s accuracy against real results, the only method to validate a marketing attribution model is through its application. Unlike these other models, marketing attribution lacks the predictive nature of true predictive models, meaning there are no “real” results to compare the model’s predictions to before launching it.
5. Get “Predictive”
In a data project, after the data is cleaned and ready, predictive models can be used. However, in marketing attribution, no predictions are made, which is why this section is titled “Get ‘Predictive’.” Rather, the result of the model will be a percentage or score assigned to each channel.
6. Visualise
Certainly, visual representations can be beneficial in demonstrating how marketing attribution works, by showing how the conversions are spread across different channels. This could be a bar graph displaying the number of conversions (or the percentage of conversions) for each channel at any given time. Alternatively, a line graph could be used to display the number of conversions per channel over time, helping to identify any changes. Changes in conversions could suggest seasonal patterns or, more commonly, unstable algorithm behaviour, indicating the need for further refinement.
7. Deploy and Iterate
Launching a marketing attribution initiative can encompass a wide range of activities, contingent upon the specific outcomes set forth by both the business and marketing departments. However, at the bare minimum, it involves implementing a model based on real data that is consistently revised with the latest information (this detail should have been established in the initial agreements with marketing — the frequency of updates may vary from daily to weekly to monthly, depending on the marketing team’s requirements and the business’s characteristics).
Marketing attribution stands out as a data science endeavour because the only way to gauge its impact is by applying the model, adjusting marketing budgets in response, and monitoring the effects on business operations. Essentially, by tweaking the model and spending, one can assess the impact on conversions — for instance, how does reducing the budget for a particular channel influence the overall conversion rate? By conducting this cycle across various channels and evaluating the outcomes on the business level, marketing departments can pinpoint the ideal equilibrium.
AI, machine learning hyper-personalisation solutions, the distinction between which is considerable, can save the ecommerce CMO enormous investment in efforts, as they have already devised facilities to do all the hard work for you. it is inherent in their facility, as it needs the data instantly to identify imminent consumer purchases and deliver a product selection unique to that consumer, instantaneously. Consequently, the data offered is in effect retrospective, as the algorithms have already used it, and probably developed further beyond that, before the reporting has been able to catch up.
Conclusion
Determining the success of advertising channels in terms of conversions is undoubtedly the most significant, yet also the most intricate, obstacle that modern marketing departments encounter.
However, there is no easy fix; while the application of data science and machine learning methods can greatly reduce the effort required and achieve superior outcomes compared to conventional rule-based approaches, it remains a process that requires ongoing attention. Marketing departments need to consistently assess the effectiveness and strategy of their channels to keep up with changes in consumer behaviour as time progresses.