Understanding Data Analytics in Ecommerce Retail. In the 2nd Quarter of 2020, US retail ecommerce sales shot up to $211.5 billion, up from $160.4 billion in the 1st Quarter. The lockdown caused by Covid-19 has certainly contributed to this boost in e-commerce sales.
The below graph looks at the Q2 e-commerce sales as a percentage of the total retail sales in the US, in the last 5 years, so that you get a good feel of the rise of the e-commerce potential.
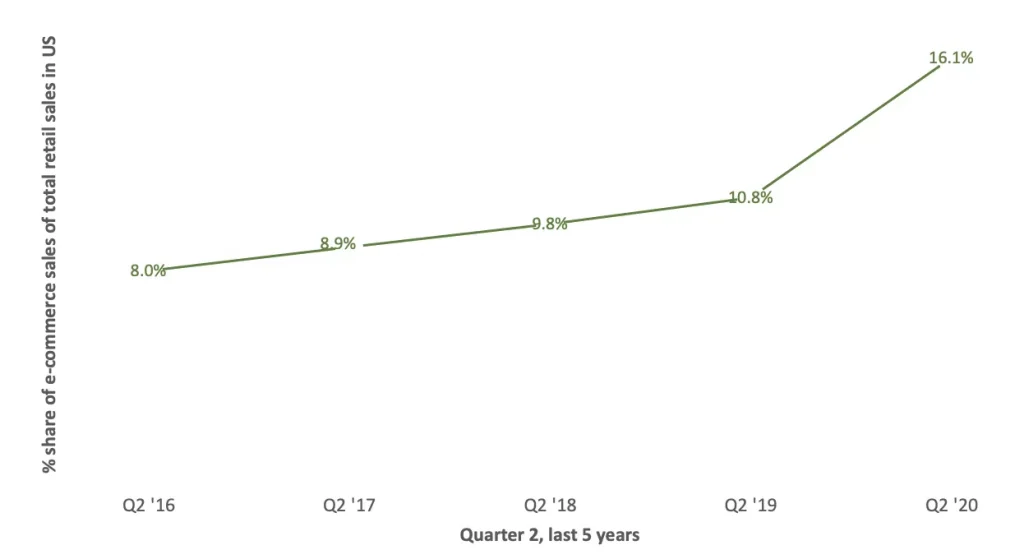
Moreover, ecommerce sales in the US are set to surpass $1 trillion to make up 18.1% of total retail sales by 2024.
Let’s take a look at the performance of the top CPG (Consumer Packaged Goods) companies so far, as compared to last year in terms of the percentage share of e-commerce sales in total retail sales.
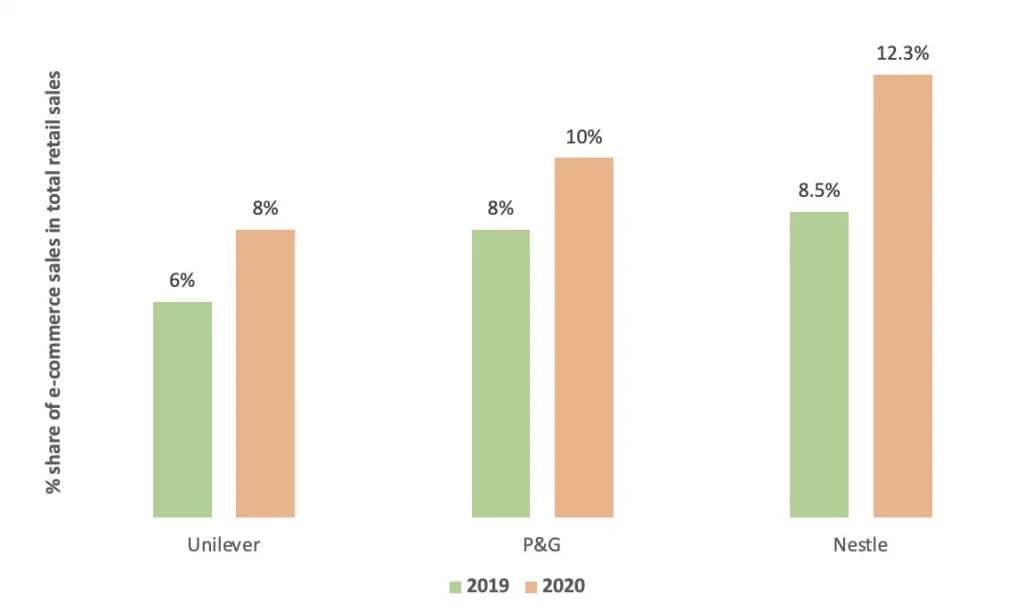
So, now you know the importance companies are given to boost their e-commerce sales.
Now, let’s dive into the different avenues where analytics has a high potential to help consumer goods companies scale up ecommerce sales.
Product Recommendations
Do you remember the time you visited the general store when the friendly assistant helped you throughout your shopping experience? He/She knew the items you bought, the preferred brands you purchased, or the style your kid loved. They knew your shopping budget and would make recommendations. And you did end up purchasing what they recommended quite a few times. Didn’t you?
That relationship with the customers is what the companies need.
A product recommendation engine is the solution. What is a recommendation engine?
It is simply an automated form of the ‘friendly assistant’. It is a tool that uses machine learning algorithms to suggest products to customers that they might be interested in. The recommendations can be made through the following channels:
- Within websites
- Email campaigns
- Online ads
The recommendation engines are of two types:
a) Impersonalised Recommendation Engine:
This is useful for sending out recommendations via welcome emails or bulk newsletters, without using customer data. Here are some useful categories:
- new products like a new chocolate ice cream that has been recently launched
- trending products like a fast-selling coffee brand to help recruit new customers
- slow-moving products like luxurious bar soap at a discounted price
b) Personalised Recommendation Engine:
This is gold! It uses customer data to recommend the best offers and promotions. It uses deep learning algorithms and Natural Language Processing (NLP) to make the customer experience as personalised as possible. The algorithms use data from the following spheres:
- Purchase history
- Recent Activity
- Online behaviour
- Social media browsing
These algorithms are really powerful and can greatly boost ecommerce sales by:
- improving conversions rates
- increasing the average purchase value of customer
- reducing bounce rates & cart abandonments. Here’s what Amazon’s recommendation engine shows currently. It looks like it knows that you love to work out.
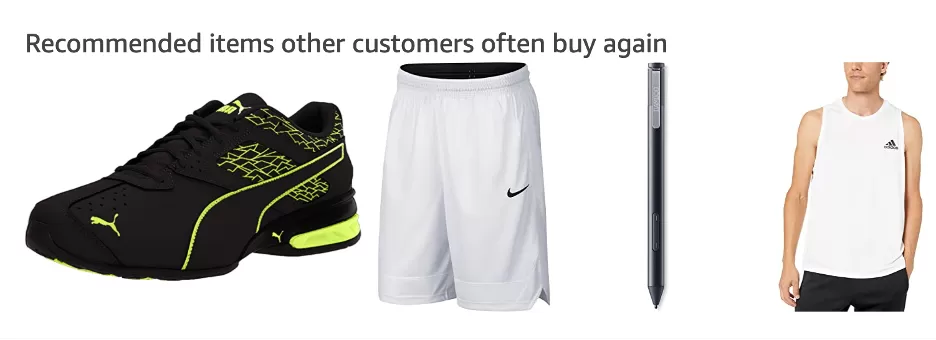
Market Basket Analysis
Market Basket Analysis is a technique that identifies the strength of association between a pair/group of products that are purchased together. In simpler words, it’s based on the concept that if a customer buys a product, they are more or less likely to buy another related product. Market Basket Analysis also uses machine learning/deep learning algorithms, like product recommendation engines.
I’ll explain this with an example from the project at Colgate-Palmolive, mentioned earlier. We bundled a children’s toothbrush (an unprofitable product) with a deodorant, and a dad’s product (a fast-moving product), and were able to increase the total weekly profit of the combination by 8%.
Association rule learning is a machine learning method that is used for discovering associations between products. It does not consider the order of the purchase of products. Let us explain this with a demonstration.
The analysis is run on millions of baskets, but we’ll demonstrate it with 7 baskets and explain the 3 important measures used. Let’s explore the association between shampoo and conditioner (Basket 1):
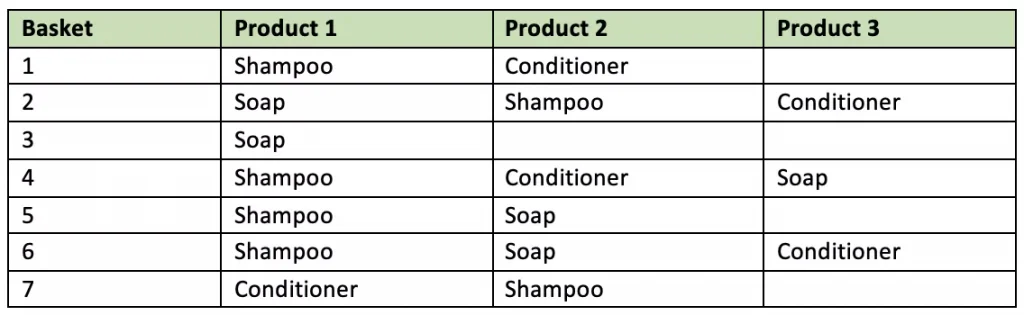
Support
Definition: No. of transactions that include both the products x and y as a percentage of the total number of transactions
Business meaning: It indicates how frequently both products are purchased together.
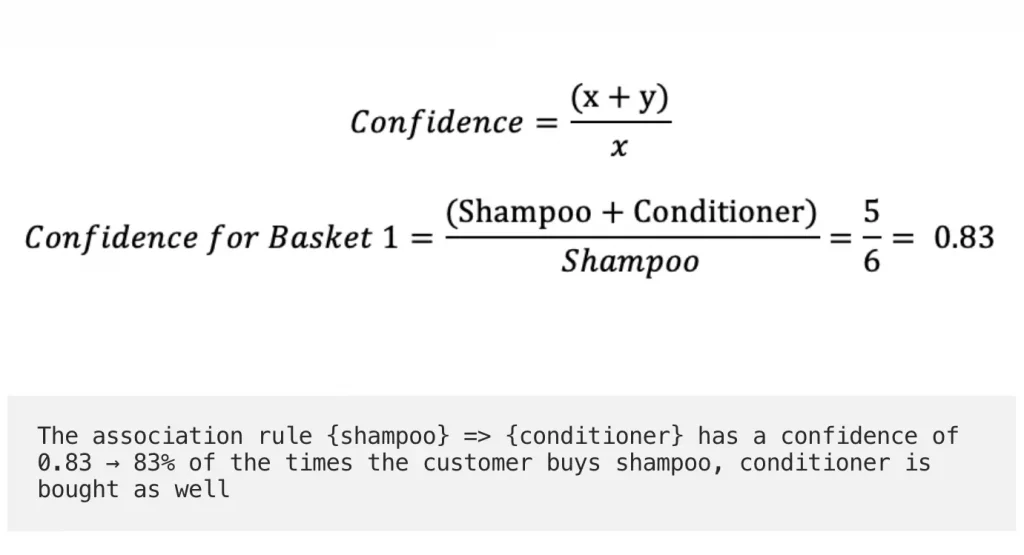
Lift
Definition:Lift is the ratio of confidence to expected confidence. Expected confidence is simply the probability that product y is in the basket.
Business meaning: The lift of a rule indicates how strong is the association within the rule, i.e. how better a rule is at predicting the result than just assuming the result in the first place. Higher lift values indicate stronger associations.
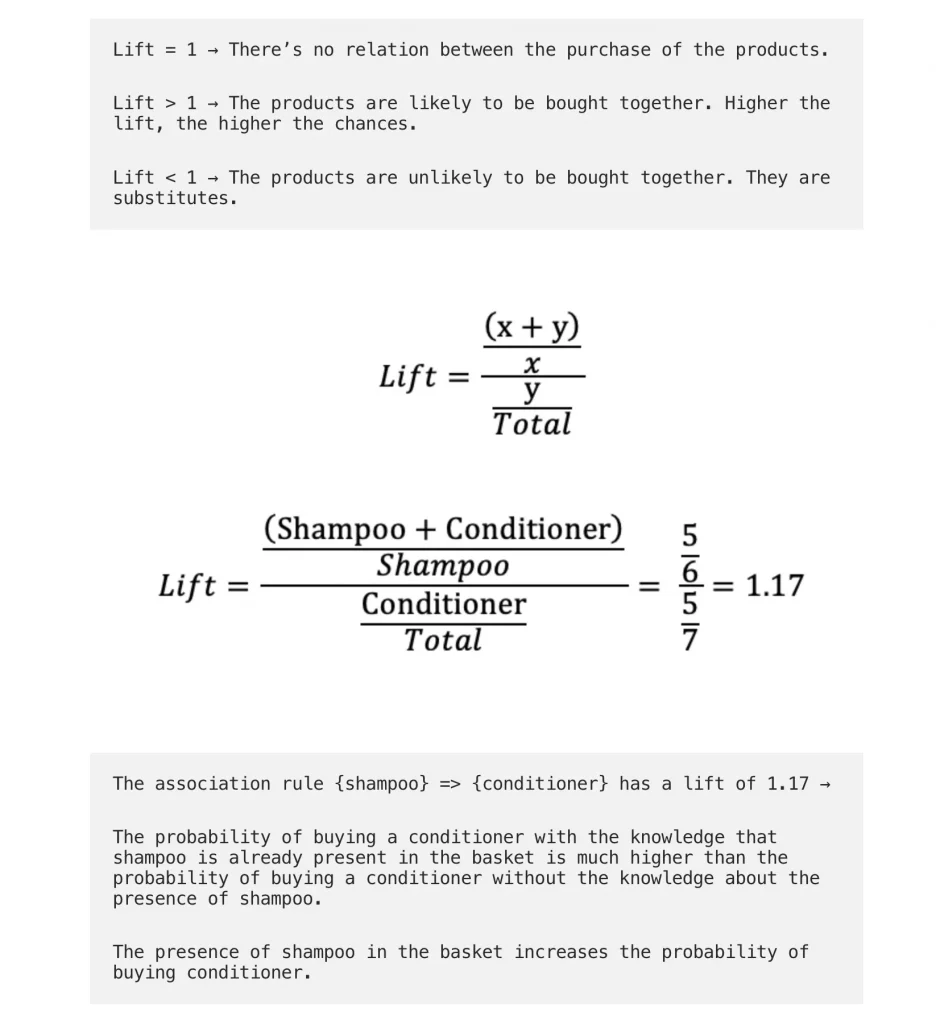
Price Optimisation
80% of people say that the most important aspect of shopping at an online ecommerce store is competitive pricing.
Segmentation of Customer & Products
This is the first step in price optimisation, where you’ll group similar products and customers. Classification (supervised) and clustering (unsupervised) algorithms are useful for this segmentation. The best technique will depend on the business requirements and the type of data.
The analysis used K-mean clustering to form groups of products with similar sales trends at Colgate. It had helped to build one predictive model per cluster instead of having many models.
Regression Modelling
Once the segmentation is done, a regression model on the various data points around sales, conversion rates, seasonality, product attributes, marketing channels etc. It helps to fix optimal prices for the products. Let’s give you a hypothetical business example from the CPG industry. The regression model built by the analytics team at Unilever for Personal Care products can help it to predict that a 5% decrease in the price of Dove deodorant can boost sales by 15%.
Dynamic Pricing
Do you remember the last time the price of your favourite product dropped during a big sale at Amazon, and the other e-commerce stores also dropped their prices?
This is dynamic pricing. It uses competitor data as input into machine learning algorithms for pricing to determine the product price at different times. This helps the products to remain viable in the competitive market with frequent price fluctuations.
A/B Testing can be used to refine the pricing model, but it needs a lot of technical considerations.
Demand Forecasting
Let’s imagine Benjamin Franklin and Abraham Lincoln were ecommerce business tycoons for a minute.
Franklin seems to have warned against underestimating the potential of demand forecasting in ecommerce retail sales,
“If you fail to plan, you are planning to fail.”
And Lincoln seems to have provided the perfect strategy for including demand forecasting in the analytics scene at your ecommerce company.
“Give me six hours to chop down a tree, and I will spend the first four sharpening the axe.”
Demand forecasting refers to the use of analytics techniques to predict the demand for a product and forecast sales. When you know the sales trends in advance, it gives you an advantage over your competitors in the following ways:
a) Better Inventory management
An underestimation of inventory levels can lead to inventory getting out of stock and hence cause customer dissatisfaction. An overestimation of inventory stock levels can lead to overstocking of inventory and hence cause unnecessary storage costs.
Demand forecasting helps in informed inventory planning and saves you from both selling out of popular products and warehouse space wastage due to slow-moving stock.
b) Better cashflow management
Since the money is not tied up in slow-moving inventory, It helps you plan the budget properly and use the cash optimally. Thus, it helps you to reduce financial risk.
c) Better pricing strategy
Effective demand forecasting also helps in improved pricing strategy. You can charge more for the products having a high demand forecast, and vice versa. You can better plan the marketing budget, advertising investment, and discount plans.
d) Better customer service
The prior knowledge of the product demands and their fluctuations helps in planning better customer service. It’s time for a business example.
Amazon patented ‘Anticipatory Shipping’ in 2013 to use predictive modelling to ship products to you before you would even make the purchase. Amazon’s algorithms would predict your purchase, and it would send the product to your nearest warehouse. Then, Amazon would wait until the actual purchase. Amazon believed that this would drastically cut the delay from the time of purchase to the actual delivery and boost the overall company sales.