Thanks to Big Data, computational leaps, and the increased availability of analytics tools, a new age of data analysis has emerged, and in the process has revolutionized the planning field. With the explosion of data and the increasing desire to leverage it as a competitive tool, companies are moving from looking in the rear-view mirror to what is in front of them – and even charting their paths.
In their book, Competing on Analytics, Thomas Davenport and Jeanne Harris describe the competitive advantage to degrees of information, or what they call intelligence. The authors divide these into two quadrants: those that are descriptive, or what I would call traditional or reactive, and those that are predictive, or what we would call revolutionary and proactive.
Building on this we can further look at the progression from pure descriptive to past predictive to prescriptive and even what some call cognitive. As we continue along, the graph allows us to see what benefits each analytics type provides see (figure 1).
Figure 1:
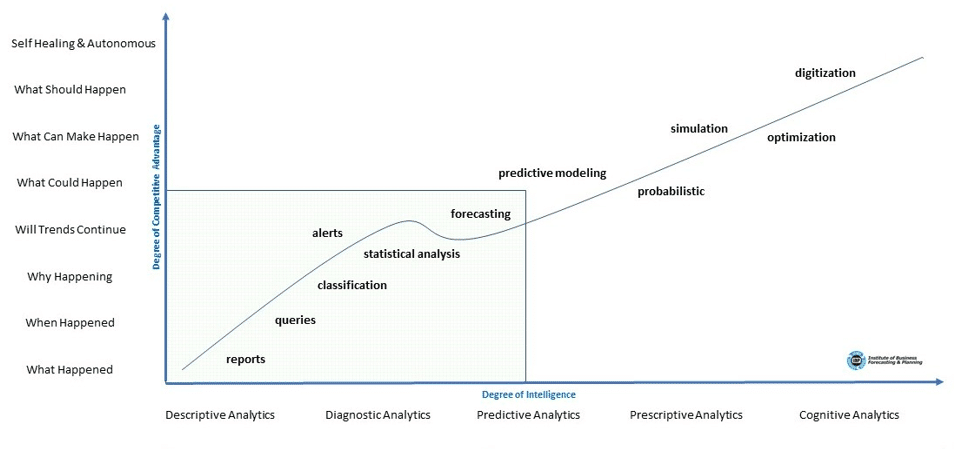
As you up the X-axis and along the Y-axis, your competitive advantage increases. But wherever your processes land on the chart, all of these processes and outputs are intended to support decision-making.
Depending on the stage of the workflow and the requirement of data analysis, there are five main kinds of analytics – descriptive, diagnostic, predictive, prescriptive and cognitive. The five types of analytics are usually implemented in stages and no one type of analytics is said to be better than the other. They are complementary and in some cases additive i.e. you cannot employ the more sophisticated analytics without using the more fundamental analytics first.
Success lies in reconciling all of these approaches within the same strategic framework. It is important to understand that all levels of analytics provide value whether it is descriptive or predictive, and all are used in different applications. That said, those who are truly leveraging analytics for competitive advantage right now are using predictive analytics, and it is this type of analytics that is driving the revolution happening today in demand planning.
Descriptive Analytics
This is the simplest stage of analytics and for this reason most organizations today use some type of descriptive analytics. The easiest way to define it is the process of gathering and interpreting data to describe what has occurred. For the most part, most reports that a business generates are descriptive and attempt to summarize historical data or try to explain why one event in the past differed from another.
In addition to reports, some queries and classification processes can fall into the category of descriptive analytics. We can use advanced machine learning algorithms at this level for more complex data mining and clustering which helps us prepare data for other types of analysis.
Descriptive analytics takes the raw data and, through data aggregation or data mining, provides valuable insights into the past. However, these findings simply signal that something is wrong or right, without explaining why. For this reason, more mature demand planning functions do not content themselves with descriptive analytics only and prefer to combine it with other types of data analytics.
Diagnostic Analytics
At this stage, you can begin to answer some of those why questions. Historical data can begin to be measured against other data to answer the question of why something happened in the past. This is the process of gathering and interpreting different data sets to identify anomalies, detect patterns, and determine relationships. Some approaches that use diagnostic analytics include alerts, drill-down, data discovery, data mining and correlations.
This can include some traditional forecasting techniques that use ratios, likelihoods and the distribution of outcomes for the analysis. Supervised machine learning training algorithms for classification and regression also fall in this type of analytics.
Most Business Intelligence stops short of this stage and is stuck in just reporting KPIs or historical data. Companies that employ seasoned demand planners go for diagnostic analytics as it gives in-depth insights into a problem and more information to support business decisions. At the same time, however, diagnostic analytics means we are reactive, and even when used in tandem with forecasting, we can only predict what existing trends may continue.
Predictive Analytics
Predictive analytics, broadly speaking, is a category of business intelligence that uses descriptive and predictive variables from the past to analyse and identify the likelihood of an unknown future outcome. It brings together several data mining methodologies, forecasting methods, predictive models and analytical techniques to analyse current data, assess risk and opportunities, capture relationships and make predictions. At this stage you are no longer just asking what happened, but why it happened, and what could happen in the future.
By successfully applying many traditional forecasting techniques to more advanced machine learning predictive algorithms, businesses can effectively interpret Big Data to gain huge competitive advantages.
Unfortunately, most companies are still only scratching the surface of the capabilities of predictive analytics and operate solely in the green-shaded area of Figure 1, stuck between “what happened” and “what could happen”. Such are the limitations of traditional business forecasting. They miss the bigger picture of predictive analytics being a new, better way to understand business. They haven’t realised that predictive analytics allows you to understand demand drivers and then use that knowledge to proactively respond to the market.
Prescriptive Analytics
Prescriptive analytics is the next step in the progression of analytics where we take:
- The data we gathered in the descriptive stage that told us what happened,
- Combine it with the diagnostic analytics that told us why it happened,
- Combine those with the predictive analytics that tell us when it may occur again.
The result is prescriptive analytics that will highlight what you can now make happen. Prescriptive analytics is a combination of data, mathematical models, and various business rules to infer actions to influence future desired outcomes. Some refer to this as demand shaping but it can also include simulation, probability maximisation and optimisation.
Prescriptive analytics is comparatively complex and many companies are not yet using them in day-to-day business activities. Admittedly, to consistently operate at this level of maturity, requires new people, processes and technology, and an analytics-driven culture for the entire organisation.
That said, if implemented properly it can have a major impact on business growth and be a competitive game-changer. Larger scale organisations like Amazon, Target and McDonald’s are already using prescriptive analytics in their demand planning to optimise customer experience and maximise sales.
Cognitive Analytics
Wouldn’t it be nice if we could take all of the analytics and data and the software learns by itself without us telling it what to do; welcome to cognitive analytics. Cognitive analytics brings together several intelligent technologies to accomplish this, including semantics, artificial intelligence algorithms and several learning techniques such as deep learning and machine learning.
Applying such techniques, a cognitive application can get smarter and self-heal and become more effective over time by learning from its interactions with data and with humans. With this, we may even begin to blur the boundary between the physical and the virtual worlds and automate processes and processing to bring new capabilities to demand planning.
5 responses
Great piece. Rarely do I encounter a blog that I truly appreciate.
Great post. I am impressed! Extremely helpful info. Thank you and good luck.
There is definitely a great deal to learn about this issue. I really like all the points you have made, thanks so much.
Bookmarked!!, I like your site!
I just spent half an hour reading your blog through my coffee-break. Brilliant stuff, thanks.