Today, data aggregators and data pools have the difficult job of compiling ecommerce data for hundreds of thousands or millions of products. This task is complicated by the fact that every product manufacturer has a different format for their product content.
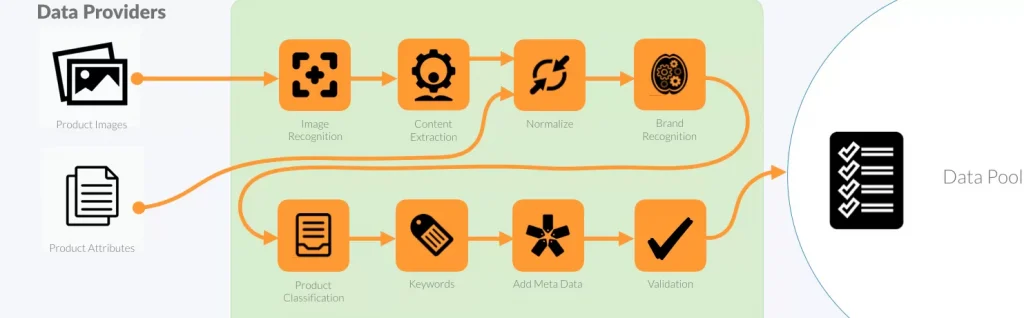
The goal of data aggregation is to consolidate product data into a form and a format that can easily be leveraged by a search engine and presented to an online buyer in a standard format. Both search engines and human users expect to see product data presented in a uniform format.
Search engines need a uniform format to improve search accuracy. Human users want to easily evaluate and compare products.
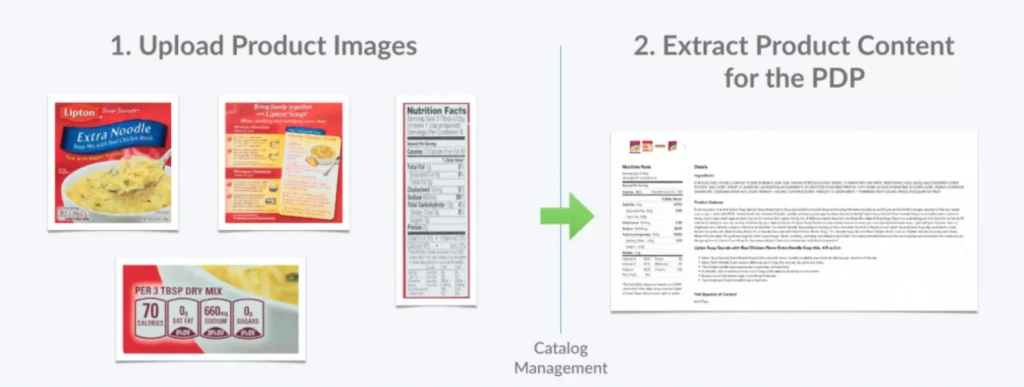
Product content can include both structured and unstructured data sources such as:
- Website content
- Manufacturer Data sheets
- Manufacturer Spec sheets
- Manufacturer Brochures
- Spreadsheets
- Product Images
- Testing or lab results
- CAD files
- PDF drawings
- Product Documentation
- User Guides
- Databases
- Product Reviews
- User Generated Content
The Ecommerce Data Consolidation Process
It is an enormous project to collect, collate, import and reconcile all of this electronic information outlined above. For the purposes of this discussion, we’ll assume that all of the content has at least been captured electronically or is available in some machine readable format.
The first step is to ingest all of the source content. For a manual ingestion process, it can take a full time dedicated staff to collect and collate all of the content. However, artificial intelligence (AI) can now help to automate the ingestion process. AI does this by understanding learning what to look for in each source file type and then extracting and organising the relevant content into a uniform output format.
AI learns what is important and what’s not important by first watching a human process the data. As a human highlights, copies and pastes the important information from a source document, the AI learns from what is important and isn’t important.
A new machine learning model is like a infant. It knows nothing. As the AI observes an operation, it can begin to learn how to extract the content on its own. Every AI machine learning model requires training data that has been tagged as the basis for learning. Generating training data can be a large part of the training process. A target data evaluation data set is also required to test the prediction accuracy of a model. A key requirement for any machine learning process is a large enough data set for training and evaluation before a model can be deployed to a production workflow process.
Automated Validation Ecommerce Data
The validation and reconciliation of information is a critical step in the data aggregation workflow. All of the information extracted must be reconciled to ensure that the source documents agree. But how do you know that the correct information was selected for reconciliation?
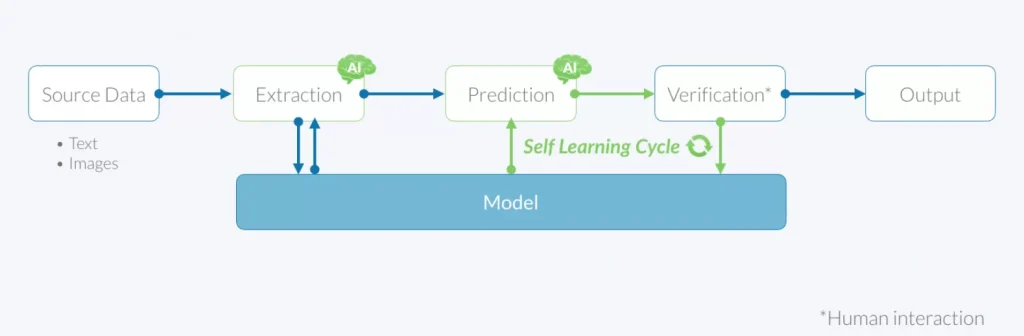
A properly engineered system can identify conflicts in the source material and either make a decision or flag the data for review. This can identify down rev’d or spurious material and force it to be rejected as irrelevant. It can also identify new information and reconcile the new data into the correct attributes. Machine learning models can help to weight the decision of which data elements are correct.
Conclusion
When a data source is unstructured data such as images, the information in the images must be properly extracted and then tested for quality. A properly trained machine learning model can automate this process. With the state of the art in AI, it’s now possible to train and deploy machine learning models using the skills of business users. Data scientists will need to be involved in the structuring of the workflow or pipeline process and the selection of machine learning models. However, AutoML is quickly evolving and it won’t be long before much of this is effected by AI decision making.
The outcome is the ability to scale AI-based systems to learn faster and operate on a broader range of data without a massive retraining cycle.